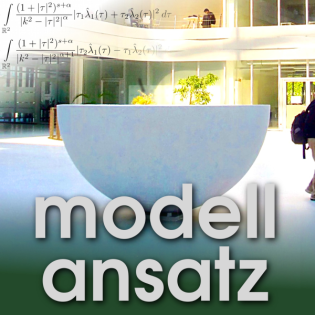
Podcast
Podcaster
Beschreibung
vor 8 Jahren
This is the last of four conversation Gudrun had during the
British Applied Mathematics Colloquium which took place 5th – 8th
April 2016 in Oxford.
Andrea Bertozzi from the University of California in Los Angeles
(UCLA) held a public lecture on The Mathematics of Crime. She has
been Professor of Mathematics at UCLA since 2003 and Betsy Wood
Knapp Chair for Innovation and Creativity (since 2012). From
1995-2004 she worked mostly at Duke University first as Associate
Professor of Mathematics and then as Professor of Mathematics and
Physics. As an undergraduate at Princeton University she studied
physics and astronomy alongside her major in mathematics and went
through a Princeton PhD-program. For her thesis she worked in
applied analysis and studied fluid flow. As postdoc she worked
with Peter Constantin at the University of Chicago (1991-1995) on
global regularity for vortex patches. But even more importantly,
this was the moment when she found research problems that needed
knowledge about PDEs and flow but in addition both numerical
analysis and scientific computing. She found out that she really
likes to collaborate with very different specialists. Today
hardwork can largely be carried out on a desktop but occasionally
clusters or supercomputers are necessary.
The initial request to work on Mathematics in crime came from a
colleague, the social scientist Jeffrey Brantingham. He works in
Anthropology at UCLA and had well established contacts with the
police in LA. He was looking for mathematical input on some of
his problems and raised that issue with Andrea Bertozzi. Her
postdoc George Mohler came up with the idea to adapt an
earthquake model after a discussion with Frederic Paik
Schoenberg, a world expert in that field working at UCLA. The
idea is to model crimes of opportunity as being triggered by
crimes that already happend. So the likelihood of new crimes can
be predicted as an excitation in space and time like the shock of
an earthquake. Of course, here statistical models are necessary
which say how the excitement is distributed and decays in space
and time. Mathematically this is a self-exciting point process.
The traditional Poisson process model has a single parameter and
thus, no memory - i.e. no connections to other events can be
modelled. The Hawkes process builds on the Poisson process as
background noise but adds new events which then are triggering
events according to an excitation rate and the exponential decay
of excitation over time. This is a memory effect based on actual
events (not only on a likelihood) and a three parameter model. It
is not too difficult to process field data, fit data to that
model and make an extrapolation in time. Meanwhile the results of
that idea work really well in the field. Results of field trials
both in the UK and US have just been published and there is a
commercial product available providing services to the police.
In addition to coming up with useful ideas and having an
interdisciplinary group of people committed to make them work it
was necessery to find funding in order to support students to
work on that topic. The first grant came from the National
Science Foundation and from this time on the group included
George Tita (UC Irvine) a criminology expert in LA-Gangs and
Lincoln Chayes as another mathematician in the team.
The practical implementation of this crime prevention method for
the police is as follows: Before the policemen go out on a shift
they ususally meet to divide their teams over the area they are
serving. The teams take the crime prediction for that shift which
is calculated by the computer model on the basis of whatever data
is available up to shift. According to expected spots of crimes
they especially assign teams to monitor those areas more closely.
After introducing this method in the police work in Santa Cruz
(California) police observed a significant reduction of 27% in
crime. Of course this is a wonderful success story. Another
success story involves the career development of the students and
postdocs who now have permanent positions. Since this was the
first group in the US to bring mathematics to police work this
opened a lot of doors for young people involved.
Another interesting topic in the context of Mathematics and crime
are gang crime data. As for the the crime prediction model the
attack of one gang on a rival gang usually triggers another event
soon afterwards. A well chosen group of undergraduates already is
mathematically educated enough to study the temporary
distribution of gang related crime in LA with 30 street gangs and
a complex net of enemies. We are speaking about hundreds of
crimes in one year related to the activity of gangs. The
mathematical tool which proved to be useful was a maximum
liklihood penalization model again for the Hawkes process applied
on the expected retaliatory behaviour.
A more complex problem, which was treated in a PhD-thesis, is to
single out gangs which would be probably responsable for certain
crimes. This means to solve the inverse problem: We know the time
and the crime and want to find out who did it. The result was
published in Inverse Problems 2011. The tool was a variational
model with an energy which is related to the data. The missing
information is guessed and then put into the energy . In finding
the best guess related to the chosen energy model a probable
candidate for the crime is found. For a small number of unsolved
crimes one can just go through all possible combinations. For
hundreds or even several hundreds of unsolved crimes - all
combinations cannot be handled. We make it easier by increasing
the number of choices and formulate a continuous instead of the
discrete problem, for which the optimization works with a
standard gradient descent algorithm.
A third topic and a third tool is Compressed sensing. It looks at
sparsitiy in data like the probability distribution for crime in
different parts of the city. Usually the crime rate is high in
certain areas of a city and very low in others. For these sharp
changes one needs different methods since we have to allow for
jumps. Here the total variation enters the model as the -norm of
the gradient. It promotes sparsity of edges in the solution.
Before coming up with this concept it was necessary to
cross-validate quite a number of times, which is computational
very expensive. So instead of in hours the result is obtained in
a couple minutes now.
When Andrea Bertozzi was a young child she spent a lot of Sundays
in the Science museum in Boston and wanted to become a scientist
when grown up. The only problem was, that she could not decide
which science would be the best choice since she liked everything
in the museum. Today she says having chosen applied mathematics
indeed she can do all science since mathematics works as a
connector between sciences and opens a lot of doors.
References
Press coverage of Crime prevention collected
Website of Mathematical and Simulation Modeling of Crime
Examples for work of undergraduates
M. Allenby, e.a.: A Point Process Model for Simulating
Gang-on-Gang Violence, Project Report, 2010.
K. Louie: Statistical Modeling of Gang Violence in Los
Angeles, talk at AMS Joint meetings San Francisco, AMS Session on
Mathematics in the Social Sciences, 2010]
Publications of A. Bertozzi and co-workers on Crime prevention
G.O. Mohler e.a.: Randomized controlled field trials of
predictive policing, J. Am. Stat. Assoc., 111(512), 1399-1411,
2015.
J. T. Woodworth e.a.: Nonlocal Crime Density Estimation
Incorporating Housing Information, Phil. Trans. Roy. Soc. A,
372(2028), 20130403, 2014.
J. Zipkin, M. B. Short & A. L. Bertozzi: Cops on the dots
in a mathematical model of urban crime and police response,
Discrete and Continuous Dynamical Systems B, 19(5), pp.
1479-1506, 2014.
H. Hu e.a.: A Method Based on Total Variation for Network
Modularity Optimization using the MBO Scheme, SIAM J. Appl.
Math., 73(6), pp. 2224-2246, 2013.
L.M. Smith e.a.: Adaptation of an Ecological Territorial
Model to Street Gang Spatial Patterns in Los Angeles Discrete and
Continuous Dynamical Systems A, 32(9), pp. 3223 - 3244, 2012.
G. Mohler e.a.. (2011): Self- exciting point process modeling
of crime, Journal of the American Statistical Association,
106(493):100–108, 2011.
A. Stomakhin, M. Short, and A. Bertozzi: Reconstruction of
missing data in social networks based on temporal patterns of
interactions. Inverse Problems, 27, 2011.
N. Rodriguez & A. Bertozzi: Local Existence and
Uniqueness of Solutions to a PDE model for Criminal Behavior ,
M3AS, special issue on Mathematics and Complexity in Human and
Life Sciences, Vol. 20, Issue supp01, pp. 1425-1457, 2010.
Related Podcasts
AMS - Mathematical Moments Podcast: MM97 - Forecasting Crime
British Applied Mathematics Colloquium 2016 Special
J.Dodd: Crop Growth, Conversation with G. Thäter in the
Modellansatz Podcast episode 89, Department of Mathematics,
Karlsruhe Institute of Technology (KIT), 2016.
http://modellansatz.de/crop-growth
H. Wilson: Viscoelastic Fluids, Conversation with G. Thäter
in the Modellansatz Podcast episode 92, Department of
Mathematics, Karlsruhe Institute of Technology (KIT), 2016.
http://modellansatz.de/viscoelastic-fluids
A. Hosoi: Robots, Conversation with G. Thäter in the
Modellansatz Podcast, episode 108, Department for Mathematics,
Karlsruhe Institute of Technologie (KIT), 2016.
http://modellansatz.de/robot
A. Bertozzi: Crime Prevention, Conversation with G. Thäter in
the Modellansatz Podcast, episode 109, Department for
Mathematics, Karlsruhe Institute of Technologie (KIT), 2016.
http://modellansatz.de/crime-prevention
Weitere Episoden

16 Minuten
vor 10 Monaten

1 Stunde 42 Minuten
vor 1 Jahr

50 Minuten
vor 2 Jahren

42 Minuten
vor 2 Jahren

35 Minuten
vor 2 Jahren
In Podcasts werben
Abonnenten
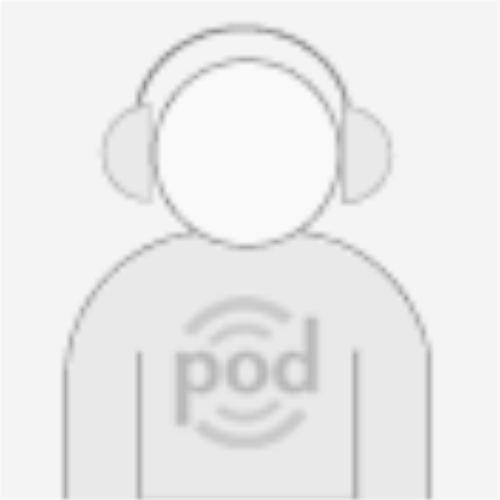
Neutraubing
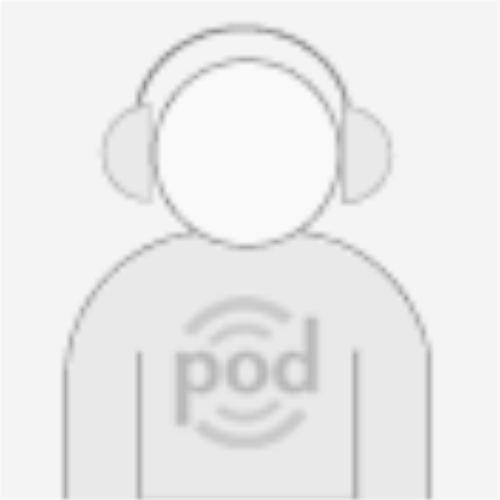
Darmstadt
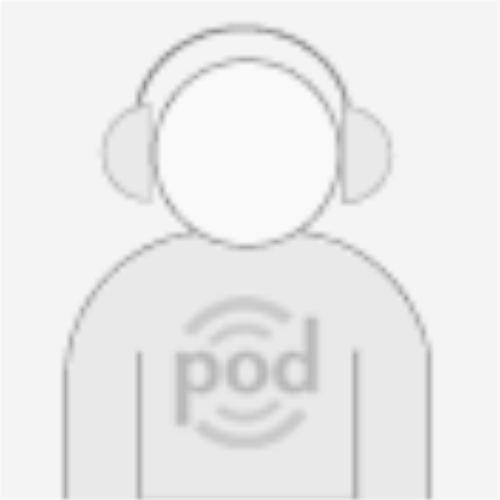
Groß Gusborn
Kommentare (0)