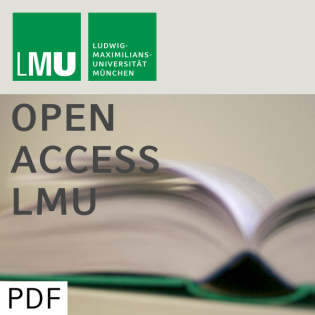
Does a Gibbs sampler approach to spatial Poisson regression models outperform a single site MH sampler?
Beschreibung
vor 19 Jahren
In this paper we present and evaluate a Gibbs sampler for a Poisson
regression model including spatial e ects. The approach is based on
Frühwirth-Schnatter and Wagner (2004b) who show that by data
augmentation using the introduction of two sequences of latent
variables a Poisson regression model can be transformed into an
approximate normal linear model. We show how this methodology can
be extended to spatial Poisson regression models and give details
of the resulting Gibbs sampler. In particular, the influence of
model parameterisation and di erent update strategies on the mixing
of the MCMC chains is discussed. The developed Gibbs samplers are
analysed in two simulation studies and applied to model the
expected number of claims for policyholders of a German car
insurance company. The mixing of the Gibbs samplers depends
crucially on the model parameterisation and the update schemes. The
best mixing is achieved when collapsed algorithms are used,
reasonable low autocorrelations for the spatial e ects are obtained
in this case. For the regression e ects however, autocorrelations
are rather high, especially for data with very low heterogeneity.
For comparison a single component Metropolis Hastings algorithms is
applied which displays very good mixing for all components.
Although the Metropolis Hastings sampler requires a higher
computational e ort, it outperforms the Gibbs samplers which would
have to be run considerably longer in order to obtain the same
precision of the parameters.
regression model including spatial e ects. The approach is based on
Frühwirth-Schnatter and Wagner (2004b) who show that by data
augmentation using the introduction of two sequences of latent
variables a Poisson regression model can be transformed into an
approximate normal linear model. We show how this methodology can
be extended to spatial Poisson regression models and give details
of the resulting Gibbs sampler. In particular, the influence of
model parameterisation and di erent update strategies on the mixing
of the MCMC chains is discussed. The developed Gibbs samplers are
analysed in two simulation studies and applied to model the
expected number of claims for policyholders of a German car
insurance company. The mixing of the Gibbs samplers depends
crucially on the model parameterisation and the update schemes. The
best mixing is achieved when collapsed algorithms are used,
reasonable low autocorrelations for the spatial e ects are obtained
in this case. For the regression e ects however, autocorrelations
are rather high, especially for data with very low heterogeneity.
For comparison a single component Metropolis Hastings algorithms is
applied which displays very good mixing for all components.
Although the Metropolis Hastings sampler requires a higher
computational e ort, it outperforms the Gibbs samplers which would
have to be run considerably longer in order to obtain the same
precision of the parameters.
Weitere Episoden



vor 11 Jahren
In Podcasts werben
Kommentare (0)