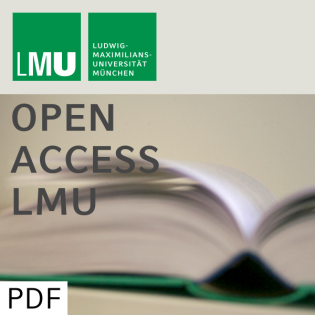
Clustering in linear mixed models with a group fused lasso penalty
Beschreibung
vor 12 Jahren
A method is proposed that aims at identifying clusters of
individuals that show similar patterns when observed repeatedly. We
consider linear mixed models which are widely used for the modeling
of longitudinal data. In contrast to the classical assumption of a
normal distribution for the random effects a finite mixture of
normal distributions is assumed. Typically, the number of mixture
components is unknown and has to be chosen, ideally by data driven
tools. For this purpose an EM algorithm-based approach is
considered that uses a penalized normal mixture as random effects
distribution. The penalty term shrinks the pairwise distances of
cluster centers based on the group lasso and the fused lasso
method. The effect is that individuals with similar time trends are
merged into the same cluster. The strength of regularization is
determined by one penalization parameter. For finding the optimal
penalization parameter a new model choice criterion is proposed.
individuals that show similar patterns when observed repeatedly. We
consider linear mixed models which are widely used for the modeling
of longitudinal data. In contrast to the classical assumption of a
normal distribution for the random effects a finite mixture of
normal distributions is assumed. Typically, the number of mixture
components is unknown and has to be chosen, ideally by data driven
tools. For this purpose an EM algorithm-based approach is
considered that uses a penalized normal mixture as random effects
distribution. The penalty term shrinks the pairwise distances of
cluster centers based on the group lasso and the fused lasso
method. The effect is that individuals with similar time trends are
merged into the same cluster. The strength of regularization is
determined by one penalization parameter. For finding the optimal
penalization parameter a new model choice criterion is proposed.
Weitere Episoden



vor 11 Jahren
In Podcasts werben
Kommentare (0)