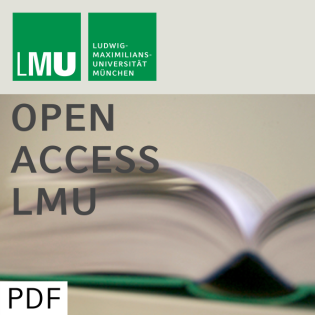
Generalized structured additive regression based on Bayesian P-splines
Beschreibung
vor 21 Jahren
Generalized additive models (GAM) for modelling nonlinear effects
of continuous covariates are now well established tools for the
applied statistician. In this paper we develop Bayesian GAM's and
extensions to generalized structured additive regression based on
one or two dimensional P-splines as the main building block. The
approach extends previous work by Lang und Brezger (2003) for
Gaussian responses. Inference relies on Markov chain Monte Carlo
(MCMC) simulation techniques, and is either based on iteratively
weighted least squares (IWLS) proposals or on latent utility
representations of (multi)categorical regression models. Our
approach covers the most common univariate response distributions,
e.g. the Binomial, Poisson or Gamma distribution, as well as
multicategorical responses. For the first time, we present Bayesian
semiparametric inference for the widely used multinomial logit
models. As we will demonstrate through two applications on the
forest health status of trees and a space-time analysis of health
insurance data, the approach allows realistic modelling of complex
problems. We consider the enormous flexibility and extendability of
our approach as a main advantage of Bayesian inference based on
MCMC techniques compared to more traditional approaches. Software
for the methodology presented in the paper is provided within the
public domain package BayesX.
of continuous covariates are now well established tools for the
applied statistician. In this paper we develop Bayesian GAM's and
extensions to generalized structured additive regression based on
one or two dimensional P-splines as the main building block. The
approach extends previous work by Lang und Brezger (2003) for
Gaussian responses. Inference relies on Markov chain Monte Carlo
(MCMC) simulation techniques, and is either based on iteratively
weighted least squares (IWLS) proposals or on latent utility
representations of (multi)categorical regression models. Our
approach covers the most common univariate response distributions,
e.g. the Binomial, Poisson or Gamma distribution, as well as
multicategorical responses. For the first time, we present Bayesian
semiparametric inference for the widely used multinomial logit
models. As we will demonstrate through two applications on the
forest health status of trees and a space-time analysis of health
insurance data, the approach allows realistic modelling of complex
problems. We consider the enormous flexibility and extendability of
our approach as a main advantage of Bayesian inference based on
MCMC techniques compared to more traditional approaches. Software
for the methodology presented in the paper is provided within the
public domain package BayesX.
Weitere Episoden



vor 11 Jahren
In Podcasts werben
Kommentare (0)