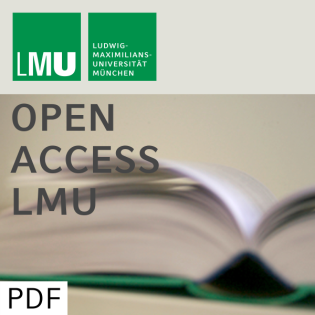
Theoretical Foundations of Autoregressive Models for Time Series on Acyclic Directed Graphs
Beschreibung
vor 21 Jahren
Three classes of models for time series on acyclic directed graphs
are considered. At first a review of tree-structured models
constructed from a nested partitioning of the observation interval
is given. This nested partitioning leads to several resolution
scales. The concept of mass balance allowing to interpret the
average over an interval as the sum of averages over the
sub-intervals implies linear restrictions in the tree-structured
model. Under a white noise assumption for transition and
observation noise there is an change-of-resolution Kalman filter
for linear least squares prediction of interval averages
\shortcite{chou:1991}. This class of models is generalized by
modeling transition noise on the same scale in linear state space
form. The third class deals with models on a more general class of
directed acyclic graphs where nodes are allowed to have two
parents. We show that these models have a linear state space
representation with white system and coloured observation noise.
are considered. At first a review of tree-structured models
constructed from a nested partitioning of the observation interval
is given. This nested partitioning leads to several resolution
scales. The concept of mass balance allowing to interpret the
average over an interval as the sum of averages over the
sub-intervals implies linear restrictions in the tree-structured
model. Under a white noise assumption for transition and
observation noise there is an change-of-resolution Kalman filter
for linear least squares prediction of interval averages
\shortcite{chou:1991}. This class of models is generalized by
modeling transition noise on the same scale in linear state space
form. The third class deals with models on a more general class of
directed acyclic graphs where nodes are allowed to have two
parents. We show that these models have a linear state space
representation with white system and coloured observation noise.
Weitere Episoden



vor 11 Jahren
In Podcasts werben
Kommentare (0)