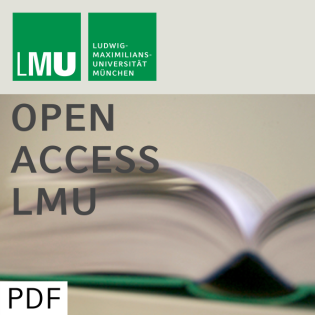
Structured count data regression
Beschreibung
vor 21 Jahren
Overdispersion in count data regression is often caused by
neglection or inappropriate modelling of individual heterogeneity,
temporal or spatial correlation, and nonlinear covariate effects.
In this paper, we develop and study semiparametric count data
models which can deal with these issues by incorporating
corresponding components in structured additive form into the
predictor. The models are fully Bayesian and inference is carried
out by computationally efficient MCMC techniques. In a simulation
study, we investigate how well the different components can be
identified with the data at hand. The approach is applied to a
large data set of claim frequencies from car insurance.
neglection or inappropriate modelling of individual heterogeneity,
temporal or spatial correlation, and nonlinear covariate effects.
In this paper, we develop and study semiparametric count data
models which can deal with these issues by incorporating
corresponding components in structured additive form into the
predictor. The models are fully Bayesian and inference is carried
out by computationally efficient MCMC techniques. In a simulation
study, we investigate how well the different components can be
identified with the data at hand. The approach is applied to a
large data set of claim frequencies from car insurance.
Weitere Episoden



vor 11 Jahren
In Podcasts werben
Kommentare (0)