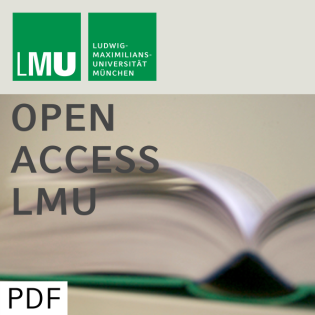
Aggregating Classifiers With Ordinal Response Structure
Beschreibung
vor 21 Jahren
In recent years the introduction of aggregation methods led to many
new techniques within the field of prediction and classification.
The most important developments, bagging and boosting, have been
extensively analyzed for two and multi class problems. While the
proposed methods treat the class indicator as a nominal response
without any structure, in many applications the class may be
considered as an ordered categorical variable. In the present paper
variants of bagging and boosting are proposed which make use of the
ordinal structure. It is demonstrated how the predictive power is
improved by use of appropriate aggregation methods. Comparisons
between the methods are based on misclassification rates as well as
criteria that take ordinality into account, like absolute or
squared distance measures.
new techniques within the field of prediction and classification.
The most important developments, bagging and boosting, have been
extensively analyzed for two and multi class problems. While the
proposed methods treat the class indicator as a nominal response
without any structure, in many applications the class may be
considered as an ordered categorical variable. In the present paper
variants of bagging and boosting are proposed which make use of the
ordinal structure. It is demonstrated how the predictive power is
improved by use of appropriate aggregation methods. Comparisons
between the methods are based on misclassification rates as well as
criteria that take ordinality into account, like absolute or
squared distance measures.
Weitere Episoden



vor 11 Jahren
In Podcasts werben
Kommentare (0)