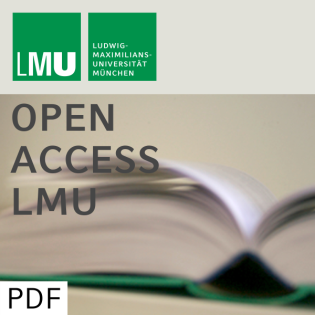
Structured additive regression for multicategorical space-time data: A mixed model approach
Beschreibung
vor 20 Jahren
In many practical situations, simple regression models suffer from
the fact that the dependence of responses on covariates can not be
sufficiently described by a purely parametric predictor. For
example effects of continuous covariates may be nonlinear or
complex interactions between covariates may be present. A specific
problem of space-time data is that observations are in general
spatially and/or temporally correlated. Moreover, unobserved
heterogeneity between individuals or units may be present. While,
in recent years, there has been a lot of work in this area dealing
with univariate response models, only limited attention has been
given to models for multicategorical space-time data. We propose a
general class of structured additive regression models (STAR) for
multicategorical responses, allowing for a flexible semiparametric
predictor. This class includes models for multinomial responses
with unordered categories as well as models for ordinal responses.
Non-linear effects of continuous covariates, time trends and
interactions between continuous covariates are modelled through
Bayesian versions of penalized splines and flexible seasonal
components. Spatial effects can be estimated based on Markov random
fields, stationary Gaussian random fields or two-dimensional
penalized splines. We present our approach from a Bayesian
perspective, allowing to treat all functions and effects within a
unified general framework by assigning appropriate priors with
different forms and degrees of smoothness. Inference is performed
on the basis of a multicategorical linear mixed model
representation. This can be viewed as posterior mode estimation and
is closely related to penalized likelihood estimation in a
frequentist setting. Variance components, corresponding to inverse
smoothing parameters, are then estimated by using restricted
maximum likelihood. Numerically efficient algorithms allow
computations even for fairly large data sets. As a typical example
we present results on an analysis of data from a forest health
survey.
the fact that the dependence of responses on covariates can not be
sufficiently described by a purely parametric predictor. For
example effects of continuous covariates may be nonlinear or
complex interactions between covariates may be present. A specific
problem of space-time data is that observations are in general
spatially and/or temporally correlated. Moreover, unobserved
heterogeneity between individuals or units may be present. While,
in recent years, there has been a lot of work in this area dealing
with univariate response models, only limited attention has been
given to models for multicategorical space-time data. We propose a
general class of structured additive regression models (STAR) for
multicategorical responses, allowing for a flexible semiparametric
predictor. This class includes models for multinomial responses
with unordered categories as well as models for ordinal responses.
Non-linear effects of continuous covariates, time trends and
interactions between continuous covariates are modelled through
Bayesian versions of penalized splines and flexible seasonal
components. Spatial effects can be estimated based on Markov random
fields, stationary Gaussian random fields or two-dimensional
penalized splines. We present our approach from a Bayesian
perspective, allowing to treat all functions and effects within a
unified general framework by assigning appropriate priors with
different forms and degrees of smoothness. Inference is performed
on the basis of a multicategorical linear mixed model
representation. This can be viewed as posterior mode estimation and
is closely related to penalized likelihood estimation in a
frequentist setting. Variance components, corresponding to inverse
smoothing parameters, are then estimated by using restricted
maximum likelihood. Numerically efficient algorithms allow
computations even for fairly large data sets. As a typical example
we present results on an analysis of data from a forest health
survey.
Weitere Episoden



vor 11 Jahren
In Podcasts werben
Kommentare (0)