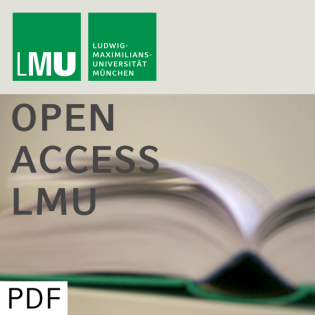
Predicting Transcription Factor Activities from Combined Analysis of Microarray and ChIP Data: A Partial Least Squares Approach
Beschreibung
vor 19 Jahren
The study of the network between transcription factors and their
targets is important for understanding the complex regulatory
mechanisms in a cell. However, due to post-translational
modifications the regulator transcription levels (as measured,
e.g., by microarray expression arrays) generally provide only
little information about the true transcription factor activities
(TFAs). Here we propose an approach based on partial least squares
(PLS) regression to infer true TFAs from expression data integrated
with information from DNA-protein binding experiments (e.g., ChIP).
This method is statistically sound also for a small number of
samples and enables to detect functional interaction among the
transcription factors themselves via the inference of
'meta'-transcription factors. In addition, it allows to identify
false positives in ChIP data as well as to predict activation and
suppression activities (which is not possible from ChIP data
alone). Subsequent to PLS inference, the estimated transcription
factor activities may be subject to further analysis such as tests
of periodicity or differential regulation. This method overcomes
the limitations of previously used approaches, and is illustrated
by analyzing expression and ChIP data from Yeast and E.Coli
experiments.
targets is important for understanding the complex regulatory
mechanisms in a cell. However, due to post-translational
modifications the regulator transcription levels (as measured,
e.g., by microarray expression arrays) generally provide only
little information about the true transcription factor activities
(TFAs). Here we propose an approach based on partial least squares
(PLS) regression to infer true TFAs from expression data integrated
with information from DNA-protein binding experiments (e.g., ChIP).
This method is statistically sound also for a small number of
samples and enables to detect functional interaction among the
transcription factors themselves via the inference of
'meta'-transcription factors. In addition, it allows to identify
false positives in ChIP data as well as to predict activation and
suppression activities (which is not possible from ChIP data
alone). Subsequent to PLS inference, the estimated transcription
factor activities may be subject to further analysis such as tests
of periodicity or differential regulation. This method overcomes
the limitations of previously used approaches, and is illustrated
by analyzing expression and ChIP data from Yeast and E.Coli
experiments.
Weitere Episoden



vor 11 Jahren
In Podcasts werben
Kommentare (0)