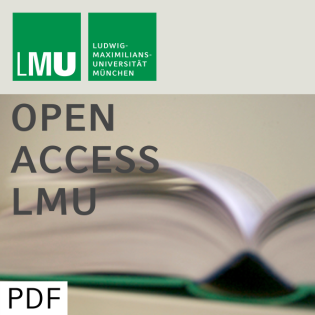
Geoadditive survival models
Beschreibung
vor 19 Jahren
Survival data often contain small-area geographical or spatial
information, such as the residence of individuals. In many cases
the impact of such spatial effects on hazard rates is of
considerable substantive interest. Therefore, extensions of known
survival or hazard rate models to spatial models have been
suggested recently. Mostly, a spatial component is added to the
usual linear predictor of the Cox model. We propose flexible
continuous-time geoadditive models, extending the Cox model with
respect to several aspects often needed in applications: The common
linear predictor is generalized to an additive predictor, including
nonparametric components for the log-baseline hazard, time-varying
effects and possibly nonlinear effects of continuous covariates or
further time scales, and a spatial component for geographical
effects. In addition, uncorrelated frailty effects or nonlinear
two-way interactions can be incorporated. Inference is developed
within a unified fully Bayesian framework. We prefer to use
penalized regression splines and Markov random fields as basic
building blocks, but geostatistical (kriging) models are also
considered. Posterior analysis uses computationally efficient MCMC
sampling schemes. Smoothing parameters are an integral part of the
model and are estimated automatically. Propriety of posteriors is
shown under fairly general conditions, and practical performance is
investigated through simulation studies. We apply our approach to
data from a case study in London and Essex that aims to estimate
the effect of area of residence and further covariates on waiting
times to coronary artery bypass graft (CABG). Results provide clear
evidence of nonlinear time-varying effects, and considerable
spatial variability of waiting times to bypass graft.
information, such as the residence of individuals. In many cases
the impact of such spatial effects on hazard rates is of
considerable substantive interest. Therefore, extensions of known
survival or hazard rate models to spatial models have been
suggested recently. Mostly, a spatial component is added to the
usual linear predictor of the Cox model. We propose flexible
continuous-time geoadditive models, extending the Cox model with
respect to several aspects often needed in applications: The common
linear predictor is generalized to an additive predictor, including
nonparametric components for the log-baseline hazard, time-varying
effects and possibly nonlinear effects of continuous covariates or
further time scales, and a spatial component for geographical
effects. In addition, uncorrelated frailty effects or nonlinear
two-way interactions can be incorporated. Inference is developed
within a unified fully Bayesian framework. We prefer to use
penalized regression splines and Markov random fields as basic
building blocks, but geostatistical (kriging) models are also
considered. Posterior analysis uses computationally efficient MCMC
sampling schemes. Smoothing parameters are an integral part of the
model and are estimated automatically. Propriety of posteriors is
shown under fairly general conditions, and practical performance is
investigated through simulation studies. We apply our approach to
data from a case study in London and Essex that aims to estimate
the effect of area of residence and further covariates on waiting
times to coronary artery bypass graft (CABG). Results provide clear
evidence of nonlinear time-varying effects, and considerable
spatial variability of waiting times to bypass graft.
Weitere Episoden



vor 11 Jahren
In Podcasts werben
Kommentare (0)