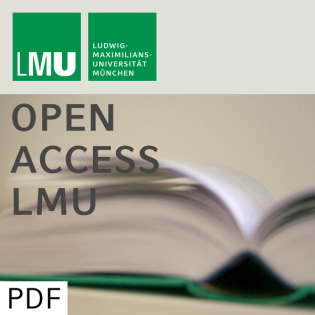
A two-component model for counts of infectious diseases
Beschreibung
vor 19 Jahren
We propose a stochastic model for the analysis of time series of
disease counts as collected in typical surveillance systems on
notifiable infectious diseases. The model is based on a Poisson or
negative binomial observation model with two components: A
parameterdriven component relates the disease incidence to latent
parameters describing endemic seasonal patterns, which are typical
for infectious disease surveillance data. A observationdriven or
epidemic component is modeled with an autoregression on the number
of cases at the previous time points. The autoregressive parameter
is allowed to change over time according to a Bayesian changepoint
model with unknown number of changepoints. Parameter estimates are
obtained through Bayesian model averaging using Markov chain Monte
Carlo (MCMC) techniques. In analyses of simulated and real datasets
we obtain promising results.
disease counts as collected in typical surveillance systems on
notifiable infectious diseases. The model is based on a Poisson or
negative binomial observation model with two components: A
parameterdriven component relates the disease incidence to latent
parameters describing endemic seasonal patterns, which are typical
for infectious disease surveillance data. A observationdriven or
epidemic component is modeled with an autoregression on the number
of cases at the previous time points. The autoregressive parameter
is allowed to change over time according to a Bayesian changepoint
model with unknown number of changepoints. Parameter estimates are
obtained through Bayesian model averaging using Markov chain Monte
Carlo (MCMC) techniques. In analyses of simulated and real datasets
we obtain promising results.
Weitere Episoden



vor 11 Jahren
In Podcasts werben
Kommentare (0)