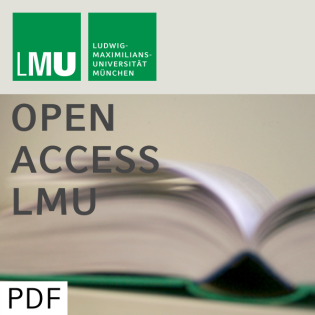
Stochastic modelling of the spatial spread of influenza in Germany
Beschreibung
vor 19 Jahren
In geographical epidemiology, disease counts are typically
available in discrete spatial units and at discrete time-points.
For example, surveillance data on infectious diseases usually
consists of weekly counts of new infections in pre-defined
geographical areas. Similarly, but on a different time-scale,
cancer registries typically report yearly incidence or mortality
counts in administrative regions. A major methodological challenge
lies in building realistic models for space-time interactions on
discrete irregular spatial graphs. In this paper, we will discuss
an observation-driven approach, where past observed counts in
neighbouring areas enter directly as explanatory variables, in
contrast to the parameter-driven approach through latent Gaussian
Markov random fields (Rue and Held, 2005) with spatio-temporal
structure. The main focus will lie on the demonstration of the
spread of influenza in Germany, obtained through the design and
simulation of a spatial extension of the classical SIR model
(Hufnagel et al., 2004).
available in discrete spatial units and at discrete time-points.
For example, surveillance data on infectious diseases usually
consists of weekly counts of new infections in pre-defined
geographical areas. Similarly, but on a different time-scale,
cancer registries typically report yearly incidence or mortality
counts in administrative regions. A major methodological challenge
lies in building realistic models for space-time interactions on
discrete irregular spatial graphs. In this paper, we will discuss
an observation-driven approach, where past observed counts in
neighbouring areas enter directly as explanatory variables, in
contrast to the parameter-driven approach through latent Gaussian
Markov random fields (Rue and Held, 2005) with spatio-temporal
structure. The main focus will lie on the demonstration of the
spread of influenza in Germany, obtained through the design and
simulation of a spatial extension of the classical SIR model
(Hufnagel et al., 2004).
Weitere Episoden



vor 11 Jahren
In Podcasts werben
Kommentare (0)