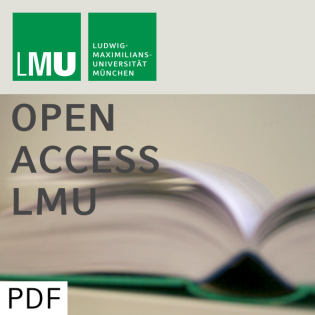
Introducing and evaluating a Gibbs sampler for spatial Poisson regression models
Beschreibung
vor 19 Jahren
In this paper we present a Gibbs sampler for a Poisson model
including spatial effects. Frühwirth-Schnatter und Wagner (2004b)
show that by data augmentation via the introduction of two
sequences of latent variables a Poisson regression model can be
transformed into a normal linear model. We show how this
methodology can be extended to spatial Poisson regression models
and give details of the resulting Gibbs sampler. In particular, the
influence of model parameterisation and different update strategies
on the mixing of the MCMC chains are discussed. The developed Gibbs
samplers are analysed in two simulation studies and appliedto model
the expected number of claims for policyholders of a German car
insurance data set. In general, both large and small simulated
spatial effects are estimated accurately by the Gibbs samplers and
reasonable low autocorrelations are obtained when the data
variability is rather large. However, for data with very low
heterogeneity, the autocorrelations resulting from the Gibbs
samplers are very high, withdrawing the computational advantage
over a Metropolis Hastings independence sampler which exhibits very
low autocorrelations in all settings.
including spatial effects. Frühwirth-Schnatter und Wagner (2004b)
show that by data augmentation via the introduction of two
sequences of latent variables a Poisson regression model can be
transformed into a normal linear model. We show how this
methodology can be extended to spatial Poisson regression models
and give details of the resulting Gibbs sampler. In particular, the
influence of model parameterisation and different update strategies
on the mixing of the MCMC chains are discussed. The developed Gibbs
samplers are analysed in two simulation studies and appliedto model
the expected number of claims for policyholders of a German car
insurance data set. In general, both large and small simulated
spatial effects are estimated accurately by the Gibbs samplers and
reasonable low autocorrelations are obtained when the data
variability is rather large. However, for data with very low
heterogeneity, the autocorrelations resulting from the Gibbs
samplers are very high, withdrawing the computational advantage
over a Metropolis Hastings independence sampler which exhibits very
low autocorrelations in all settings.
Weitere Episoden



vor 11 Jahren
In Podcasts werben
Kommentare (0)