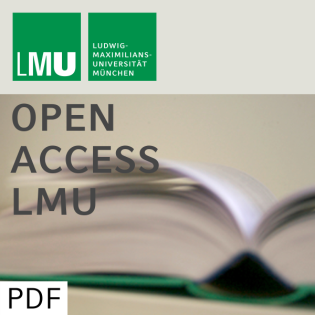
Modelling count data with overdispersion and spatial effects
Beschreibung
vor 18 Jahren
In this paper we consider regression models for count data allowing
for overdispersion in a Bayesian framework. We account for
unobserved heterogeneity in the data in two ways. On the one hand,
we consider more flexible models than a common Poisson model
allowing for overdispersion in different ways. In particular, the
negative binomial and the generalized Poisson distribution are
addressed where overdispersion is modelled by an additional model
parameter. Further, zero-inflated models in which overdispersion is
assumed to be caused by an excessive number of zeros are discussed.
On the other hand, extra spatial variability in the data is taken
into account by adding spatial random effects to the models. This
approach allows for an underlying spatial dependency structure
which is modelled using a conditional autoregressive prior based on
Pettitt et al. (2002). In an application the presented models are
used to analyse the number of invasive meningococcal disease cases
in Germany in the year 2004. Models are compared according to the
deviance information criterion (DIC) suggested by Spiegelhalter et
al. (2002) and using proper scoring rules, see for example Gneiting
and Raftery (2004). We observe a rather high degree of
overdispersion in the data which is captured best by the GP model
when spatial effects are neglected. While the addition of spatial
effects to the models allowing for overdispersion gives no or only
little improvement, a spatial Poisson model is to be preferred over
all other models according to the considered criteria.
for overdispersion in a Bayesian framework. We account for
unobserved heterogeneity in the data in two ways. On the one hand,
we consider more flexible models than a common Poisson model
allowing for overdispersion in different ways. In particular, the
negative binomial and the generalized Poisson distribution are
addressed where overdispersion is modelled by an additional model
parameter. Further, zero-inflated models in which overdispersion is
assumed to be caused by an excessive number of zeros are discussed.
On the other hand, extra spatial variability in the data is taken
into account by adding spatial random effects to the models. This
approach allows for an underlying spatial dependency structure
which is modelled using a conditional autoregressive prior based on
Pettitt et al. (2002). In an application the presented models are
used to analyse the number of invasive meningococcal disease cases
in Germany in the year 2004. Models are compared according to the
deviance information criterion (DIC) suggested by Spiegelhalter et
al. (2002) and using proper scoring rules, see for example Gneiting
and Raftery (2004). We observe a rather high degree of
overdispersion in the data which is captured best by the GP model
when spatial effects are neglected. While the addition of spatial
effects to the models allowing for overdispersion gives no or only
little improvement, a spatial Poisson model is to be preferred over
all other models according to the considered criteria.
Weitere Episoden



vor 11 Jahren
In Podcasts werben
Kommentare (0)