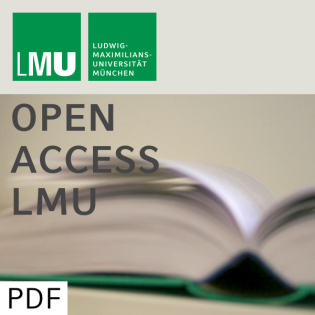
Bayesian Learning for a Class of Priors with Prescribed Marginals
Beschreibung
vor 18 Jahren
We present Bayesian updating of an imprecise probability measure,
represented by a class of precise multidimensional probability
measures. Choice and analysis of our class are motivated by expert
interviews that we conducted with modelers in the context of
climatic change. From the interviews we deduce that generically,
experts hold a much more informed opinion on the marginals of
uncertain parameters rather than on their correlations.
Accordingly, we specify the class by prescribing precise measures
for the marginals while letting the correlation structure subject
to complete ignorance. For sake of transparency, our discussion
focuses on the tutorial example of a linear two-dimensional
Gaussian model. We operationalize Bayesian learning for that class
by various updating rules, starting with (a modified version of)
the generalized Bayes' rule and the maximum likelihood update rule
(after Gilboa and Schmeidler). Over a large range of potential
observations, the generalized Bayes' rule would provide
non-informative results. We restrict this counter-intuitive and
unnecessary growth of uncertainty by two means, the discussion of
which refers to any kind of imprecise model, not only to our class.
First, we find our class of priors too inclusive and, hence,
require certain additional properties of prior measures in terms of
smoothness of probability density functions. Second, we argue that
both updating rules are dissatisfying, the generalized Bayes' rule
being too conservative, i.e., too inclusive, the maximum likelihood
rule being too exclusive. Instead, we introduce two new ways of
Bayesian updating of imprecise probabilities: a ``weighted maximum
likelihood method'' and a ``semi-classical method.'' The former
bases Bayesian updating on the whole set of priors, however, with
weighted influence of its members. By referring to the whole set,
the weighted maximum likelihood method allows for more robust
inferences than the standard maximum likelihood method and, hence,
is better to justify than the latter.Furthermore, the
semi-classical method is more objective than the weighted maximum
likelihood method as it does not require the subjective definition
of a weighting function. Both new methods reveal much more
informative results than the generalized Bayes' rule, what we
demonstrate for the example of a stylized insurance model.
represented by a class of precise multidimensional probability
measures. Choice and analysis of our class are motivated by expert
interviews that we conducted with modelers in the context of
climatic change. From the interviews we deduce that generically,
experts hold a much more informed opinion on the marginals of
uncertain parameters rather than on their correlations.
Accordingly, we specify the class by prescribing precise measures
for the marginals while letting the correlation structure subject
to complete ignorance. For sake of transparency, our discussion
focuses on the tutorial example of a linear two-dimensional
Gaussian model. We operationalize Bayesian learning for that class
by various updating rules, starting with (a modified version of)
the generalized Bayes' rule and the maximum likelihood update rule
(after Gilboa and Schmeidler). Over a large range of potential
observations, the generalized Bayes' rule would provide
non-informative results. We restrict this counter-intuitive and
unnecessary growth of uncertainty by two means, the discussion of
which refers to any kind of imprecise model, not only to our class.
First, we find our class of priors too inclusive and, hence,
require certain additional properties of prior measures in terms of
smoothness of probability density functions. Second, we argue that
both updating rules are dissatisfying, the generalized Bayes' rule
being too conservative, i.e., too inclusive, the maximum likelihood
rule being too exclusive. Instead, we introduce two new ways of
Bayesian updating of imprecise probabilities: a ``weighted maximum
likelihood method'' and a ``semi-classical method.'' The former
bases Bayesian updating on the whole set of priors, however, with
weighted influence of its members. By referring to the whole set,
the weighted maximum likelihood method allows for more robust
inferences than the standard maximum likelihood method and, hence,
is better to justify than the latter.Furthermore, the
semi-classical method is more objective than the weighted maximum
likelihood method as it does not require the subjective definition
of a weighting function. Both new methods reveal much more
informative results than the generalized Bayes' rule, what we
demonstrate for the example of a stylized insurance model.
Weitere Episoden



vor 11 Jahren
In Podcasts werben
Kommentare (0)