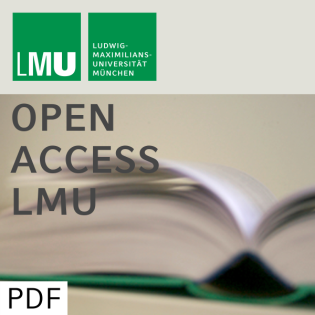
Multiresolution Analysis of Long Time Series with Applications to Finance
Beschreibung
vor 19 Jahren
We consider multi-resolution time series models and their
application to high-frequency financial data. An individual
transaction share price of a specific firm is subject to market
microstructure noise. Therefore, we propose trading duration time
weighted averages over given time intervals. Averages over long
intervals lead to a coarse resolution and averaging over shorter
intervals lead to a finer resolution. Arranging sub-intervals of
given lengths on scales with coarse to fine resolution imply a
structure which can be represented as a directed acyclic graph.
Time series models are then formulated using this graph structure.
It is shown that these models have a linear state space
representation which allows for efficient computation of the
likelihood needed in parameter estimation and for a straightforward
treatment of missing observations. Application of these models to
the log transaction prices of the IBM shares traded at the New York
Stock Exchange from February until October 2002 show that the
corresponding one-step prediction errors are heavy tailed and
therefore a specific variance term is allowed to follow a fiEGARCH
specification, improving the tail behavior and leading to a better
fit.
application to high-frequency financial data. An individual
transaction share price of a specific firm is subject to market
microstructure noise. Therefore, we propose trading duration time
weighted averages over given time intervals. Averages over long
intervals lead to a coarse resolution and averaging over shorter
intervals lead to a finer resolution. Arranging sub-intervals of
given lengths on scales with coarse to fine resolution imply a
structure which can be represented as a directed acyclic graph.
Time series models are then formulated using this graph structure.
It is shown that these models have a linear state space
representation which allows for efficient computation of the
likelihood needed in parameter estimation and for a straightforward
treatment of missing observations. Application of these models to
the log transaction prices of the IBM shares traded at the New York
Stock Exchange from February until October 2002 show that the
corresponding one-step prediction errors are heavy tailed and
therefore a specific variance term is allowed to follow a fiEGARCH
specification, improving the tail behavior and leading to a better
fit.
Weitere Episoden



vor 12 Jahren
In Podcasts werben
Kommentare (0)