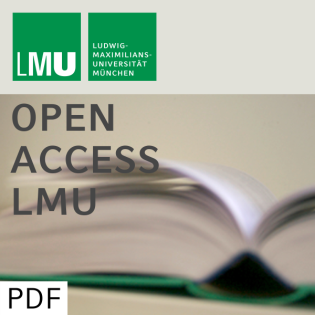
Bayesian Semiparametric Multi-State Models
Beschreibung
vor 18 Jahren
Multi-state models provide a unified framework for the description
of the evolution of discrete phenomena in continuous time. One
particular example are Markov processes which can be characterised
by a set of time-constant transition intensities between the
states. In this paper, we will extend such parametric approaches to
semiparametric models with flexible transition intensities based on
Bayesian versions of penalised splines. The transition intensities
will be modelled as smooth functions of time and can further be
related to parametric as well as nonparametric covariate effects.
Covariates with time-varying effects and frailty terms can be
included in addition. Inference will be conducted either fully
Bayesian using Markov chain Monte Carlo simulation techniques or
empirically Bayesian based on a mixed model representation. A
counting process representation of semiparametric multi-state
models provides the likelihood formula and also forms the basis for
model validation via martingale residual processes. As an
application, we will consider human sleep data with a discrete set
of sleep states such as REM and Non-REM phases. In this case,
simple parametric approaches are inappropriate since the dynamics
underlying human sleep are strongly varying throughout the night
and individual-specific variation has to be accounted for using
covariate information and frailty terms.
of the evolution of discrete phenomena in continuous time. One
particular example are Markov processes which can be characterised
by a set of time-constant transition intensities between the
states. In this paper, we will extend such parametric approaches to
semiparametric models with flexible transition intensities based on
Bayesian versions of penalised splines. The transition intensities
will be modelled as smooth functions of time and can further be
related to parametric as well as nonparametric covariate effects.
Covariates with time-varying effects and frailty terms can be
included in addition. Inference will be conducted either fully
Bayesian using Markov chain Monte Carlo simulation techniques or
empirically Bayesian based on a mixed model representation. A
counting process representation of semiparametric multi-state
models provides the likelihood formula and also forms the basis for
model validation via martingale residual processes. As an
application, we will consider human sleep data with a discrete set
of sleep states such as REM and Non-REM phases. In this case,
simple parametric approaches are inappropriate since the dynamics
underlying human sleep are strongly varying throughout the night
and individual-specific variation has to be accounted for using
covariate information and frailty terms.
Weitere Episoden



vor 11 Jahren
In Podcasts werben
Kommentare (0)