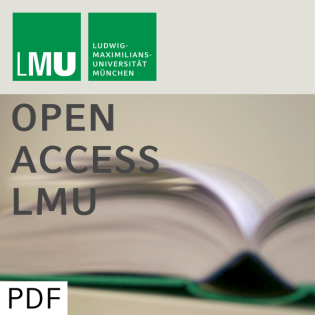
A geoadditive Bayesian latent variable model for Poisson indicators
Beschreibung
vor 18 Jahren
We introduce a new latent variable model with count variable
indicators, where usual linear parametric effects of covariates,
nonparametric effects of continuous covariates and spatial effects
on the continuous latent variables are modelled through a
geoadditive predictor. Bayesian modelling of nonparametric
functions and spatial effects is based on penalized spline and
Markov random field priors. Full Bayesian inference is performed
via an auxiliary variable Gibbs sampling technique, using a recent
suggestion of Frühwirth-Schnatter and Wagner (2006). As an
advantage, our Poisson indicator latent variable model can be
combined with semiparametric latent variable models for mixed
binary, ordinal and continuous indicator variables within an
unified and coherent framework for modelling and inference. A
simulation study investigates performance, and an application to
post war human security in Cambodia illustrates the approach.
indicators, where usual linear parametric effects of covariates,
nonparametric effects of continuous covariates and spatial effects
on the continuous latent variables are modelled through a
geoadditive predictor. Bayesian modelling of nonparametric
functions and spatial effects is based on penalized spline and
Markov random field priors. Full Bayesian inference is performed
via an auxiliary variable Gibbs sampling technique, using a recent
suggestion of Frühwirth-Schnatter and Wagner (2006). As an
advantage, our Poisson indicator latent variable model can be
combined with semiparametric latent variable models for mixed
binary, ordinal and continuous indicator variables within an
unified and coherent framework for modelling and inference. A
simulation study investigates performance, and an application to
post war human security in Cambodia illustrates the approach.
Weitere Episoden



vor 11 Jahren
In Podcasts werben
Kommentare (0)