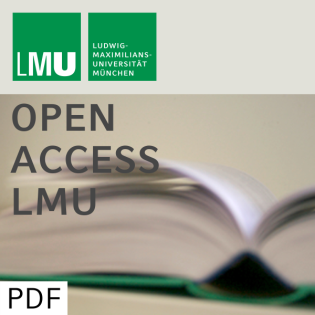
Modeling dependencies between rating categories and their effects on prediction in a credit risk portfolio
Beschreibung
vor 19 Jahren
The internal-ratings based Basel II approach increases the need for
the development of more realistic default probability models. In
this paper we follow the approach taken in McNeil and Wendin (2006)
by constructing generalized linear mixed models for estimating
default probabilities from annual data on companies with different
credit ratings. The models considered, in contrast to McNeil and
Wendin (2006), allow parsimonious parametric models to capture
simultaneously dependencies of the default probabilities on time
and credit ratings. Macro-economic variables can also be included.
Estimation of all model parameters are facilitated with a Bayesian
approach using Markov Chain Monte Carlo methods. Special emphasis
is given to the investigation of predictive capabilities of the
models considered. In particular predictable model specifications
are used. The empirical study using default data from Standard and
Poor gives evidence that the correlation between credit ratings
further apart decreases and is higher than the one induced by the
autoregressive time dynamics.
the development of more realistic default probability models. In
this paper we follow the approach taken in McNeil and Wendin (2006)
by constructing generalized linear mixed models for estimating
default probabilities from annual data on companies with different
credit ratings. The models considered, in contrast to McNeil and
Wendin (2006), allow parsimonious parametric models to capture
simultaneously dependencies of the default probabilities on time
and credit ratings. Macro-economic variables can also be included.
Estimation of all model parameters are facilitated with a Bayesian
approach using Markov Chain Monte Carlo methods. Special emphasis
is given to the investigation of predictive capabilities of the
models considered. In particular predictable model specifications
are used. The empirical study using default data from Standard and
Poor gives evidence that the correlation between credit ratings
further apart decreases and is higher than the one induced by the
autoregressive time dynamics.
Weitere Episoden



vor 12 Jahren
In Podcasts werben
Kommentare (0)