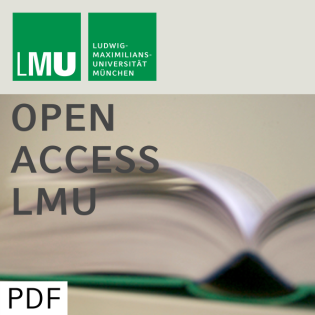
Modeling gene expression measurement error: a quasi-likelihood approach
Podcast
Podcaster
Beschreibung
vor 21 Jahren
Background: Using suitable error models for gene expression
measurements is essential in the statistical analysis of microarray
data. However, the true probabilistic model underlying gene
expression intensity readings is generally not known. Instead, in
currently used approaches some simple parametric model is assumed
(usually a transformed normal distribution) or the empirical
distribution is estimated. However, both these strategies may not
be optimal for gene expression data, as the non-parametric approach
ignores known structural information whereas the fully parametric
models run the risk of misspecification. A further related problem
is the choice of a suitable scale for the model (e. g. observed vs.
log-scale). Results: Here a simple semi-parametric model for gene
expression measurement error is presented. In this approach
inference is based an approximate likelihood function (the extended
quasi-likelihood). Only partial knowledge about the unknown true
distribution is required to construct this function. In case of
gene expression this information is available in the form of the
postulated (e.g. quadratic) variance structure of the data. As the
quasi-likelihood behaves (almost) like a proper likelihood, it
allows for the estimation of calibration and variance parameters,
and it is also straightforward to obtain corresponding approximate
confidence intervals. Unlike most other frameworks, it also allows
analysis on any preferred scale, i.e. both on the original linear
scale as well as on a transformed scale. It can also be employed in
regression approaches to model systematic (e.g. array or dye)
effects. Conclusions: The quasi-likelihood framework provides a
simple and versatile approach to analyze gene expression data that
does not make any strong distributional assumptions about the
underlying error model. For several simulated as well as real data
sets it provides a better fit to the data than competing models. In
an example it also improved the power of tests to identify
differential expression.
measurements is essential in the statistical analysis of microarray
data. However, the true probabilistic model underlying gene
expression intensity readings is generally not known. Instead, in
currently used approaches some simple parametric model is assumed
(usually a transformed normal distribution) or the empirical
distribution is estimated. However, both these strategies may not
be optimal for gene expression data, as the non-parametric approach
ignores known structural information whereas the fully parametric
models run the risk of misspecification. A further related problem
is the choice of a suitable scale for the model (e. g. observed vs.
log-scale). Results: Here a simple semi-parametric model for gene
expression measurement error is presented. In this approach
inference is based an approximate likelihood function (the extended
quasi-likelihood). Only partial knowledge about the unknown true
distribution is required to construct this function. In case of
gene expression this information is available in the form of the
postulated (e.g. quadratic) variance structure of the data. As the
quasi-likelihood behaves (almost) like a proper likelihood, it
allows for the estimation of calibration and variance parameters,
and it is also straightforward to obtain corresponding approximate
confidence intervals. Unlike most other frameworks, it also allows
analysis on any preferred scale, i.e. both on the original linear
scale as well as on a transformed scale. It can also be employed in
regression approaches to model systematic (e.g. array or dye)
effects. Conclusions: The quasi-likelihood framework provides a
simple and versatile approach to analyze gene expression data that
does not make any strong distributional assumptions about the
underlying error model. For several simulated as well as real data
sets it provides a better fit to the data than competing models. In
an example it also improved the power of tests to identify
differential expression.
Weitere Episoden

vor 19 Jahren



In Podcasts werben
Kommentare (0)