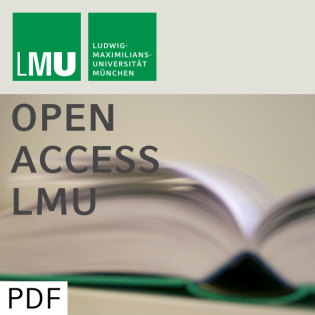
Estimation with Numerical Integration on Sparse Grids
Podcast
Podcaster
Beschreibung
vor 19 Jahren
For the estimation of many econometric models, integrals without
analytical solutions have to be evaluated. Examples include limited
dependent variables and nonlinear panel data models. In the case of
one-dimensional integrals, Gaussian quadrature is known to work
efficiently for a large class of problems. In higher dimensions,
similar approaches discussed in the literature are either very
specific and hard to implement or suffer from exponentially rising
computational costs in the number of dimensions - a problem known
as the "curse of dimensionality" of numerical integration. We
propose a strategy that shares the advantages of Gaussian
quadrature methods, is very general and easily implemented, and
does not suffer from the curse of dimensionality. Monte Carlo
experiments for the random parameters logit model indicate the
superior performance of the proposed method over simulation
techniques.
analytical solutions have to be evaluated. Examples include limited
dependent variables and nonlinear panel data models. In the case of
one-dimensional integrals, Gaussian quadrature is known to work
efficiently for a large class of problems. In higher dimensions,
similar approaches discussed in the literature are either very
specific and hard to implement or suffer from exponentially rising
computational costs in the number of dimensions - a problem known
as the "curse of dimensionality" of numerical integration. We
propose a strategy that shares the advantages of Gaussian
quadrature methods, is very general and easily implemented, and
does not suffer from the curse of dimensionality. Monte Carlo
experiments for the random parameters logit model indicate the
superior performance of the proposed method over simulation
techniques.
Weitere Episoden

vor 18 Jahren

vor 19 Jahren

vor 19 Jahren
In Podcasts werben
Abonnenten

"Estimation with Numerical Integration on Sparse Grids"
"Volkswirtschaft - Open Access LMU - Teil 01/03"
15
15
00:00
00:00
"Estimation with Numerical Integration on Sparse Grids"
"Volkswirtschaft - Open Access LMU - Teil 01/03"
Kommentare (0)