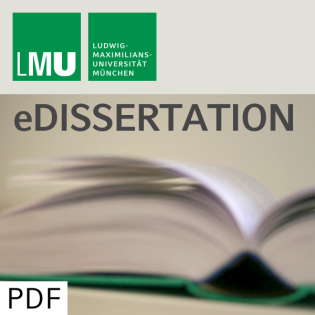
Measurement Error in Exposure Assessment: An Error Model and its Impact on Studies on Lung Cancer and Residential Radon Exposure in Germany
Beschreibung
vor 22 Jahren
Case-control studies on lung cancer and residential radon exposure
had been conducted in Germany. Relative risk estimates from primary
analysis were now subject to accounting for uncertainties in radon
exposure and in the most potent confounder smoking. The regression
calibration method and an approximate maximum likelihood method
were applied. The differentiation between classical error (from
assessing radon exposure or packyears) or Berkson error (from using
radon exposure instead of alpha dose, from using packyears instead
of inhaled dose of smoking carcinogens) was of major importance in
this analysis. Estimates of relative lung cancer risk due to radon
exposure were found to be higher after accounting for
multiplicative classical error in radon exposure and packyears.
Outliers in the data strongly influence risk estimates, but their
impact is reduced, if classical error is accounted for. In one
study, the influence of one outlier explained the particularly
large risk-increasing impact of error correction. But also residual
confounding due to adjusting for imprecisely measured packyears
deflated the risk estimate in this study. It is interesting that
the small correlation between radon exposure and packyears had this
notable effect. On the other hand, classical errors in packyears
had no large impact in the radon-prone study areas. Further,
Berkson error did not induce substantial bias on the radon risk
estimates, but possibly decreased the power to detect existing
effects and inflated the confidence intervals. It was concluded
that such an analysis was extremely valuable to understand the
impact of uncertainties in the risk factor of primary interest on
the risk estimate under study and the potential for residual
confounding by assessment errors in the smoking variable. Note that
assuming some error in the risk factors is more realistic than
assuming no error. With regard to study design, study regions with
no correlation between the variable of primary interest and
potential confounders are preferable. However, the exact magnitude
of the error could not be estimated based on the available data.
Further investigations regarding residual confounding due to model
mis-specification and latent smoking-related variables are
necessary to grasp the full dimension of an important issue in
epidemiology, i.e. the role of the outstanding confounder smoking
for estimating small risks.
had been conducted in Germany. Relative risk estimates from primary
analysis were now subject to accounting for uncertainties in radon
exposure and in the most potent confounder smoking. The regression
calibration method and an approximate maximum likelihood method
were applied. The differentiation between classical error (from
assessing radon exposure or packyears) or Berkson error (from using
radon exposure instead of alpha dose, from using packyears instead
of inhaled dose of smoking carcinogens) was of major importance in
this analysis. Estimates of relative lung cancer risk due to radon
exposure were found to be higher after accounting for
multiplicative classical error in radon exposure and packyears.
Outliers in the data strongly influence risk estimates, but their
impact is reduced, if classical error is accounted for. In one
study, the influence of one outlier explained the particularly
large risk-increasing impact of error correction. But also residual
confounding due to adjusting for imprecisely measured packyears
deflated the risk estimate in this study. It is interesting that
the small correlation between radon exposure and packyears had this
notable effect. On the other hand, classical errors in packyears
had no large impact in the radon-prone study areas. Further,
Berkson error did not induce substantial bias on the radon risk
estimates, but possibly decreased the power to detect existing
effects and inflated the confidence intervals. It was concluded
that such an analysis was extremely valuable to understand the
impact of uncertainties in the risk factor of primary interest on
the risk estimate under study and the potential for residual
confounding by assessment errors in the smoking variable. Note that
assuming some error in the risk factors is more realistic than
assuming no error. With regard to study design, study regions with
no correlation between the variable of primary interest and
potential confounders are preferable. However, the exact magnitude
of the error could not be estimated based on the available data.
Further investigations regarding residual confounding due to model
mis-specification and latent smoking-related variables are
necessary to grasp the full dimension of an important issue in
epidemiology, i.e. the role of the outstanding confounder smoking
for estimating small risks.
Weitere Episoden


In Podcasts werben
Kommentare (0)