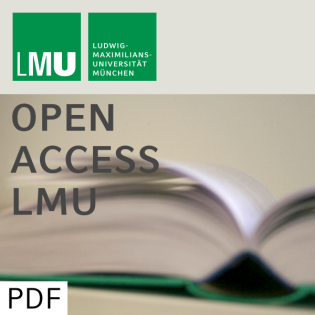
Alternative regression models to assess increase in childhood BMI
Podcast
Podcaster
Beschreibung
vor 16 Jahren
Background: Body mass index (BMI) data usually have skewed
distributions, for which common statistical modeling approaches
such as simple linear or logistic regression have limitations.
Methods: Different regression approaches to predict childhood BMI
by goodness-of-fit measures and means of interpretation were
compared including generalized linear models (GLMs), quantile
regression and Generalized Additive Models for Location, Scale and
Shape (GAMLSS). We analyzed data of 4967 children participating in
the school entry health examination in Bavaria, Germany, from 2001
to 2002. TV watching, meal frequency, breastfeeding, smoking in
pregnancy, maternal obesity, parental social class and weight gain
in the first 2 years of life were considered as risk factors for
obesity. Results: GAMLSS showed a much better fit regarding the
estimation of risk factors effects on transformed and untransformed
BMI data than common GLMs with respect to the generalized Akaike
information criterion. In comparison with GAMLSS, quantile
regression allowed for additional interpretation of prespecified
distribution quantiles, such as quantiles referring to overweight
or obesity. The variables TV watching, maternal BMI and weight gain
in the first 2 years were directly, and meal frequency was
inversely significantly associated with body composition in any
model type examined. In contrast, smoking in pregnancy was not
directly, and breastfeeding and parental social class were not
inversely significantly associated with body composition in GLM
models, but in GAMLSS and partly in quantile regression models.
Risk factor specific BMI percentile curves could be estimated from
GAMLSS and quantile regression models. Conclusion: GAMLSS and
quantile regression seem to be more appropriate than common GLMs
for risk factor modeling of BMI data.
distributions, for which common statistical modeling approaches
such as simple linear or logistic regression have limitations.
Methods: Different regression approaches to predict childhood BMI
by goodness-of-fit measures and means of interpretation were
compared including generalized linear models (GLMs), quantile
regression and Generalized Additive Models for Location, Scale and
Shape (GAMLSS). We analyzed data of 4967 children participating in
the school entry health examination in Bavaria, Germany, from 2001
to 2002. TV watching, meal frequency, breastfeeding, smoking in
pregnancy, maternal obesity, parental social class and weight gain
in the first 2 years of life were considered as risk factors for
obesity. Results: GAMLSS showed a much better fit regarding the
estimation of risk factors effects on transformed and untransformed
BMI data than common GLMs with respect to the generalized Akaike
information criterion. In comparison with GAMLSS, quantile
regression allowed for additional interpretation of prespecified
distribution quantiles, such as quantiles referring to overweight
or obesity. The variables TV watching, maternal BMI and weight gain
in the first 2 years were directly, and meal frequency was
inversely significantly associated with body composition in any
model type examined. In contrast, smoking in pregnancy was not
directly, and breastfeeding and parental social class were not
inversely significantly associated with body composition in GLM
models, but in GAMLSS and partly in quantile regression models.
Risk factor specific BMI percentile curves could be estimated from
GAMLSS and quantile regression models. Conclusion: GAMLSS and
quantile regression seem to be more appropriate than common GLMs
for risk factor modeling of BMI data.
Weitere Episoden


In Podcasts werben
Kommentare (0)