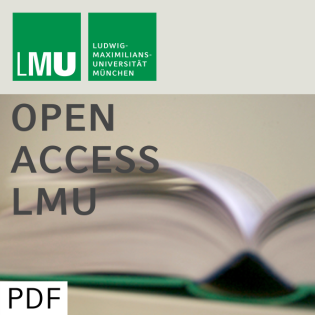
Extraction of semantic biomedical relations from text using conditional random fields
Podcast
Podcaster
Beschreibung
vor 16 Jahren
Background: The increasing amount of published literature in
biomedicine represents an immense source of knowledge, which can
only efficiently be accessed by a new generation of automated
information extraction tools. Named entity recognition of
well-defined objects, such as genes or proteins, has achieved a
sufficient level of maturity such that it can form the basis for
the next step: the extraction of relations that exist between the
recognized entities. Whereas most early work focused on the mere
detection of relations, the classification of the type of relation
is also of great importance and this is the focus of this work. In
this paper we describe an approach that extracts both the existence
of a relation and its type. Our work is based on Conditional Random
Fields, which have been applied with much success to the task of
named entity recognition. Results: We benchmark our approach on two
different tasks. The first task is the identification of semantic
relations between diseases and treatments. The available data set
consists of manually annotated PubMed abstracts. The second task is
the identification of relations between genes and diseases from a
set of concise phrases, so-called GeneRIF (Gene Reference Into
Function) phrases. In our experimental setting, we do not assume
that the entities are given, as is often the case in previous
relation extraction work. Rather the extraction of the entities is
solved as a subproblem. Compared with other state-of-the-art
approaches, we achieve very competitive results on both data sets.
To demonstrate the scalability of our solution, we apply our
approach to the complete human GeneRIF database. The resulting
gene-disease network contains 34758 semantic associations between
4939 genes and 1745 diseases. The gene-disease network is publicly
available as a machine-readable RDF graph. Conclusion: We extend
the framework of Conditional Random Fields towards the annotation
of semantic relations from text and apply it to the biomedical
domain. Our approach is based on a rich set of textual features and
achieves a performance that is competitive to leading approaches.
The model is quite general and can be extended to handle arbitrary
biological entities and relation types. The resulting gene-disease
network shows that the GeneRIF database provides a rich knowledge
source for text mining. Current work is focused on improving the
accuracy of detection of entities as well as entity boundaries,
which will also greatly improve the relation extraction
performance.
biomedicine represents an immense source of knowledge, which can
only efficiently be accessed by a new generation of automated
information extraction tools. Named entity recognition of
well-defined objects, such as genes or proteins, has achieved a
sufficient level of maturity such that it can form the basis for
the next step: the extraction of relations that exist between the
recognized entities. Whereas most early work focused on the mere
detection of relations, the classification of the type of relation
is also of great importance and this is the focus of this work. In
this paper we describe an approach that extracts both the existence
of a relation and its type. Our work is based on Conditional Random
Fields, which have been applied with much success to the task of
named entity recognition. Results: We benchmark our approach on two
different tasks. The first task is the identification of semantic
relations between diseases and treatments. The available data set
consists of manually annotated PubMed abstracts. The second task is
the identification of relations between genes and diseases from a
set of concise phrases, so-called GeneRIF (Gene Reference Into
Function) phrases. In our experimental setting, we do not assume
that the entities are given, as is often the case in previous
relation extraction work. Rather the extraction of the entities is
solved as a subproblem. Compared with other state-of-the-art
approaches, we achieve very competitive results on both data sets.
To demonstrate the scalability of our solution, we apply our
approach to the complete human GeneRIF database. The resulting
gene-disease network contains 34758 semantic associations between
4939 genes and 1745 diseases. The gene-disease network is publicly
available as a machine-readable RDF graph. Conclusion: We extend
the framework of Conditional Random Fields towards the annotation
of semantic relations from text and apply it to the biomedical
domain. Our approach is based on a rich set of textual features and
achieves a performance that is competitive to leading approaches.
The model is quite general and can be extended to handle arbitrary
biological entities and relation types. The resulting gene-disease
network shows that the GeneRIF database provides a rich knowledge
source for text mining. Current work is focused on improving the
accuracy of detection of entities as well as entity boundaries,
which will also greatly improve the relation extraction
performance.
Weitere Episoden


In Podcasts werben
Kommentare (0)