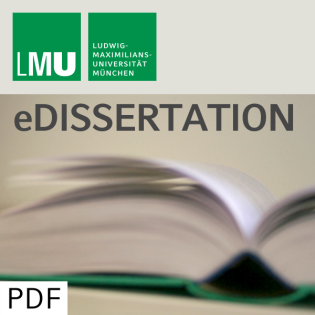
Flexible Modellierung kategorialer Responsevariablen
Beschreibung
vor 21 Jahren
Multicategorical regression models are an established tool in
statistical data analysis. The present thesis extends common
parametric regression models for nominal and ordinal responses to
more flexible nonparametric approaches. In order to obtain a
flexible form of the functional effects of metrically scaled
covariates, expansions in basis functions are used. The resulting
predictor allows parameter estimation within the framework of
multivariate generalized linear models. Estimates are obtained by
maximizing a penalized likelihood with discrete penalty terms
restricting the variation of estimated smooth effects. As a result
of theoretical considerations, P-Splines seem to be the ideal
alternative for applying penalized basis function approaches. Based
on this result, nonparametric extensions of the multinomial logit
model for nominal and the cumulative logit model for ordinal
responses are derived. An important feature of the proposed
multinomial logit model is the distinction between global and
category-specific variables. Variables of both types may enter the
model in a linear form or as unspecified smooth functions. For
cumulative logit models the penalization concept adopted from
P-Splines is used to restrict category-specific parameters in
adjacent categories. Penalization across response categories
ensures availability of estimates when common estimation procedures
fail to converge, so that tests for proportional odds may be
performed even for critical settings. Additionally, penalties
across response categories are taken into account as fixed
methodical parts when fitting semiparametric partial proportional
odds models.
statistical data analysis. The present thesis extends common
parametric regression models for nominal and ordinal responses to
more flexible nonparametric approaches. In order to obtain a
flexible form of the functional effects of metrically scaled
covariates, expansions in basis functions are used. The resulting
predictor allows parameter estimation within the framework of
multivariate generalized linear models. Estimates are obtained by
maximizing a penalized likelihood with discrete penalty terms
restricting the variation of estimated smooth effects. As a result
of theoretical considerations, P-Splines seem to be the ideal
alternative for applying penalized basis function approaches. Based
on this result, nonparametric extensions of the multinomial logit
model for nominal and the cumulative logit model for ordinal
responses are derived. An important feature of the proposed
multinomial logit model is the distinction between global and
category-specific variables. Variables of both types may enter the
model in a linear form or as unspecified smooth functions. For
cumulative logit models the penalization concept adopted from
P-Splines is used to restrict category-specific parameters in
adjacent categories. Penalization across response categories
ensures availability of estimates when common estimation procedures
fail to converge, so that tests for proportional odds may be
performed even for critical settings. Additionally, penalties
across response categories are taken into account as fixed
methodical parts when fitting semiparametric partial proportional
odds models.
Weitere Episoden

vor 11 Jahren

vor 11 Jahren

vor 11 Jahren
In Podcasts werben
Abonnenten

"Flexible Modellierung kategorialer Responsevariablen"
"Fakultät für Mathematik, Informatik und Statistik - Digitale Hochschulschriften der LMU - Teil 01/02"
15
15
00:00
00:00
"Flexible Modellierung kategorialer Responsevariablen"
"Fakultät für Mathematik, Informatik und Statistik - Digitale Hochschulschriften der LMU - Teil 01/02"
Kommentare (0)