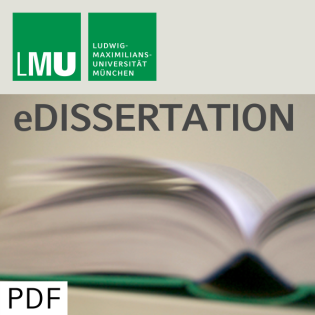
A Bayesian semiparametric latent variable model for binary, ordinal and continuous response
Beschreibung
vor 18 Jahren
This thesis discusses a latent variable model (LVM) which is based
on a Bayesian approach and is estimated by Markov chain Monte Carlo
methods (MCMC). The model extends classic factor analysis by
allowing not only for gaussian metric manifest variables, but also
for binary and ordinal indicators which are very common in many
areas of application (e.g. psychology, sociology). Furthermore, a
semiparametric predictor is introduced which describes the
influence of covariates on the latent variables. The predictor may
contain parametric effects, smooth functions of metric covariates
(modeled by random walks and P-splines), spatial effects (modeled
by Markov random fields) and interactions of metric and categorical
covariates. The integration of temporal effects is easily possible.
Consequently, the influence of covariates on the latent variables
can be analyzed in much more detail than with currently available
methods. One emphasis of this work is the development of an
efficient MCMC algorithm with good estimation properties (in
particular concerning the cutpoints of ordinal indicators) and its
implementation in the standard software package R. Another focus
lies on the demonstration of the model's applicability using data
from an internet survey. Several models with differently structured
predictors are analyzed and first ideas for model selection are
presented.
on a Bayesian approach and is estimated by Markov chain Monte Carlo
methods (MCMC). The model extends classic factor analysis by
allowing not only for gaussian metric manifest variables, but also
for binary and ordinal indicators which are very common in many
areas of application (e.g. psychology, sociology). Furthermore, a
semiparametric predictor is introduced which describes the
influence of covariates on the latent variables. The predictor may
contain parametric effects, smooth functions of metric covariates
(modeled by random walks and P-splines), spatial effects (modeled
by Markov random fields) and interactions of metric and categorical
covariates. The integration of temporal effects is easily possible.
Consequently, the influence of covariates on the latent variables
can be analyzed in much more detail than with currently available
methods. One emphasis of this work is the development of an
efficient MCMC algorithm with good estimation properties (in
particular concerning the cutpoints of ordinal indicators) and its
implementation in the standard software package R. Another focus
lies on the demonstration of the model's applicability using data
from an internet survey. Several models with differently structured
predictors are analyzed and first ideas for model selection are
presented.
Weitere Episoden

vor 11 Jahren

vor 11 Jahren

vor 11 Jahren
In Podcasts werben
Kommentare (0)