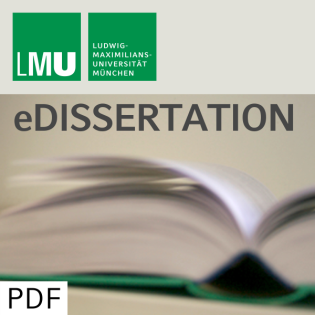
Mixed model based inference in structured additive regression
Beschreibung
vor 18 Jahren
Due to the increasing availability of spatial or spatio-temporal
regression data, models that allow to incorporate the special
structure of such data sets in an appropriate way are highly
desired in practice. A flexible modeling approach should not only
be able to account for spatial and temporal correlations, but also
to model further covariate effects in a semi- or nonparametric
fashion. In addition, regression models for different types of
responses are available and extensions require special attention in
each of these cases. Within this thesis, numerous possibilities to
model non-standard covariate effects such as nonlinear effects of
continuous covariates, temporal effects, spatial effects,
interaction effects or unobserved heterogeneity are reviewed and
embedded in the general framework of structured additive
regression. Beginning with exponential family regression,
extensions to several types of multicategorical responses and the
analysis of continuous survival times are described. A new
inferential procedure based on mixed model methodology is
introduced, allowing for a unified treatment of the different
regression problems. Estimation of the regression coefficients is
based on penalized likelihood, whereas smoothing parameters are
estimated using restricted maximum likelihood or marginal
likelihood. In several applications and simulation studies, the new
approach turns out to be a promising alternative to competing
methodology, especially estimation based on Markov Chain Monte
Carlo simulation techniques.
regression data, models that allow to incorporate the special
structure of such data sets in an appropriate way are highly
desired in practice. A flexible modeling approach should not only
be able to account for spatial and temporal correlations, but also
to model further covariate effects in a semi- or nonparametric
fashion. In addition, regression models for different types of
responses are available and extensions require special attention in
each of these cases. Within this thesis, numerous possibilities to
model non-standard covariate effects such as nonlinear effects of
continuous covariates, temporal effects, spatial effects,
interaction effects or unobserved heterogeneity are reviewed and
embedded in the general framework of structured additive
regression. Beginning with exponential family regression,
extensions to several types of multicategorical responses and the
analysis of continuous survival times are described. A new
inferential procedure based on mixed model methodology is
introduced, allowing for a unified treatment of the different
regression problems. Estimation of the regression coefficients is
based on penalized likelihood, whereas smoothing parameters are
estimated using restricted maximum likelihood or marginal
likelihood. In several applications and simulation studies, the new
approach turns out to be a promising alternative to competing
methodology, especially estimation based on Markov Chain Monte
Carlo simulation techniques.
Weitere Episoden

vor 11 Jahren

vor 11 Jahren

vor 11 Jahren
In Podcasts werben
Kommentare (0)