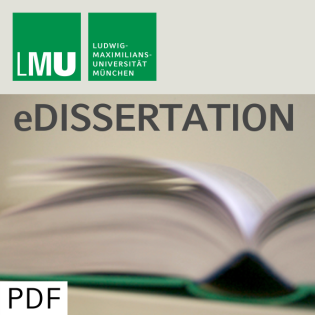
Correction for covariate measurement error in nonparametric regression
Beschreibung
vor 18 Jahren
Many areas of applied statistics have become aware of the problem
of measurement error-prone variables and their appropriate
analysis. Simply ignoring the error in the analysis usually leads
to biased estimates, like e.g. in the regression with error-prone
covariates. While this problem has been discussed at length for
parametric regression, only few methods exist to handle
nonparametric regression under error, which are usually either
computer intensive or little effective. This thesis develops new
methods achieving the correction quality of state of the art
methods while demanding only a trickle of their computing time.
These new methods use the so-called relevance vector machine (RVM)
for nonparametric regression - now enhanced by correction methods
based on the ideas of regression calibration, the so-called SIMEX
and Markov Chain Monte Carlo (MCMC) correction. All methods are
compared in simulation studies regarding Gaussian, binary and
Poisson responses. This thesis also discusses the case of multiple
error-prone covariates. Furthermore, a MCMC based correction method
for nonparametric regression of binary longitudinal data with
covariate measurement error is introduced. This data scenario is
often encountered, e.g. in epidemiological applications.
of measurement error-prone variables and their appropriate
analysis. Simply ignoring the error in the analysis usually leads
to biased estimates, like e.g. in the regression with error-prone
covariates. While this problem has been discussed at length for
parametric regression, only few methods exist to handle
nonparametric regression under error, which are usually either
computer intensive or little effective. This thesis develops new
methods achieving the correction quality of state of the art
methods while demanding only a trickle of their computing time.
These new methods use the so-called relevance vector machine (RVM)
for nonparametric regression - now enhanced by correction methods
based on the ideas of regression calibration, the so-called SIMEX
and Markov Chain Monte Carlo (MCMC) correction. All methods are
compared in simulation studies regarding Gaussian, binary and
Poisson responses. This thesis also discusses the case of multiple
error-prone covariates. Furthermore, a MCMC based correction method
for nonparametric regression of binary longitudinal data with
covariate measurement error is introduced. This data scenario is
often encountered, e.g. in epidemiological applications.
Weitere Episoden

vor 11 Jahren

vor 11 Jahren

vor 11 Jahren
In Podcasts werben
Kommentare (0)