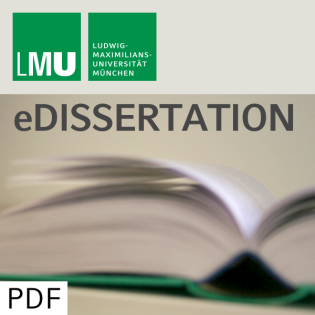
Statistical Models for Infectious Disease Surveillance Counts
Beschreibung
vor 17 Jahren
Models for infectious disease surveillance counts have to take into
account the specific characteristics of this type of data. While
showing a regular, often seasonal, pattern over long time periods,
there are occasional irregularities or outbreaks. A model which is
a compromise between mechanistic models and empirical models is
proposed. A key idea is to distinguish between an endemic and an
epidemic component, which allows to separate the regular pattern
from the irregularities and outbreaks. This is of particular
advantage for outbreak detection in public health surveillance.
While the endemic component is parameter-driven, the epidemic
component is based on observationdriven approaches, including an
autoregression on past observations. A particular challenge of
infectious disease counts is the modelling of the outbreaks and
irregularities in the data. We model the autoregressive parameter
of the epidemic component by a Bayesian changepoint model, which
shows an adaptive amount of smoothing, and is able to model the
jumps and fast increases as well as the smooth decreases in the
data. While the model can be used as a generic approach for
infectious disease counts, it is particularly suited for outbreak
detection in public health surveillance. Furthermore, the
predictive qualities of the Bayesian changepoint model allow for
short term predictions of the number of disease cases, which are of
particular public health interest. A sequential update using a
particle filter is provided, that can be used for a prospective
analysis of the changepoint model conditioning on fixed values for
the other parameters, which is of particular advantage for public
health surveillance. A suitable multivariate extension is provided,
that is able to explain the interactions between units, e.g. age
groups or spatial regions. An application to influenza and
meningococcal disease data shows that the occasional outbreaks of
meningococcal disease can largely be explained by the influence of
influenza on meningococcal disease. The risk of a future
meningococcal disease outbreak caused by influenza can be
predicted. The comparison of the different models, including a
model based on Gaussian Markov random fields shows that the
inclusion of the epidemic component as well as a time varying
epidemic parameter improves the fit and the predictive qualities of
the model.
account the specific characteristics of this type of data. While
showing a regular, often seasonal, pattern over long time periods,
there are occasional irregularities or outbreaks. A model which is
a compromise between mechanistic models and empirical models is
proposed. A key idea is to distinguish between an endemic and an
epidemic component, which allows to separate the regular pattern
from the irregularities and outbreaks. This is of particular
advantage for outbreak detection in public health surveillance.
While the endemic component is parameter-driven, the epidemic
component is based on observationdriven approaches, including an
autoregression on past observations. A particular challenge of
infectious disease counts is the modelling of the outbreaks and
irregularities in the data. We model the autoregressive parameter
of the epidemic component by a Bayesian changepoint model, which
shows an adaptive amount of smoothing, and is able to model the
jumps and fast increases as well as the smooth decreases in the
data. While the model can be used as a generic approach for
infectious disease counts, it is particularly suited for outbreak
detection in public health surveillance. Furthermore, the
predictive qualities of the Bayesian changepoint model allow for
short term predictions of the number of disease cases, which are of
particular public health interest. A sequential update using a
particle filter is provided, that can be used for a prospective
analysis of the changepoint model conditioning on fixed values for
the other parameters, which is of particular advantage for public
health surveillance. A suitable multivariate extension is provided,
that is able to explain the interactions between units, e.g. age
groups or spatial regions. An application to influenza and
meningococcal disease data shows that the occasional outbreaks of
meningococcal disease can largely be explained by the influence of
influenza on meningococcal disease. The risk of a future
meningococcal disease outbreak caused by influenza can be
predicted. The comparison of the different models, including a
model based on Gaussian Markov random fields shows that the
inclusion of the epidemic component as well as a time varying
epidemic parameter improves the fit and the predictive qualities of
the model.
Weitere Episoden

vor 11 Jahren

vor 11 Jahren

vor 11 Jahren
In Podcasts werben
Kommentare (0)