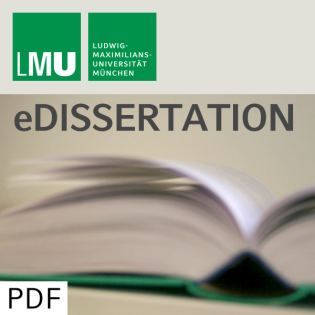
Modelling extreme wind speeds
Beschreibung
vor 17 Jahren
Very strong wind gusts can cause derailment of some high speed
trains so knowledge of the wind process at extreme levels is
required. Since the sensitivity of the train to strong wind
occurrences varies with the relative direction of a gust this
aspect has to be accounted for. We first focus on the wind process
at one weather station. An extreme value model accounting at the
same time for very strong wind speeds and wind directions is
considered and applied to both raw data and component data, where
the latter represent the force of the wind in a chosen direction.
Extreme quantiles and exceedance probabilities are estimated and we
give corresponding confidence intervals. A common problem with wind
data, called the masking problem, is that per time interval only
the largest wind speed over all directions is recorded, while
occurrences in all other directions remain unrecorded for this time
interval. To improve model estimates we suggest a model accounting
for the masking problem. A simulation study is carried out to
analyse the behaviour of this model under different conditions; the
performance is judged by comparing the new model with a traditional
model using the mean square error of high quantiles. Thereafter the
model is applied to wind data. The model turns out to have
desirable properties in the simulation study as well as in the data
application. We further consider a multivariate extreme value model
recently introduced; it allows for a broad range of dependence
structures and is thus ideally suited for many applications. As the
dependence structure of this model is characterised by several
components, quantifying the degree of dependence is not straight
forward. We therefore consider visual summary measures to support
judging the degree of dependence and study their behaviour and
usefulness via a simulation study. Subsequently, the new
multivariate extreme value model is applied to wind data of two
gauging stations where directional aspects are accounted for.
Therefore this model allows for statements about the joint wind
behaviour at the two stations. This knowledge gives insight whether
storm events are likely to be jointly present at larger parts of a
railway track or rather occur localized.
trains so knowledge of the wind process at extreme levels is
required. Since the sensitivity of the train to strong wind
occurrences varies with the relative direction of a gust this
aspect has to be accounted for. We first focus on the wind process
at one weather station. An extreme value model accounting at the
same time for very strong wind speeds and wind directions is
considered and applied to both raw data and component data, where
the latter represent the force of the wind in a chosen direction.
Extreme quantiles and exceedance probabilities are estimated and we
give corresponding confidence intervals. A common problem with wind
data, called the masking problem, is that per time interval only
the largest wind speed over all directions is recorded, while
occurrences in all other directions remain unrecorded for this time
interval. To improve model estimates we suggest a model accounting
for the masking problem. A simulation study is carried out to
analyse the behaviour of this model under different conditions; the
performance is judged by comparing the new model with a traditional
model using the mean square error of high quantiles. Thereafter the
model is applied to wind data. The model turns out to have
desirable properties in the simulation study as well as in the data
application. We further consider a multivariate extreme value model
recently introduced; it allows for a broad range of dependence
structures and is thus ideally suited for many applications. As the
dependence structure of this model is characterised by several
components, quantifying the degree of dependence is not straight
forward. We therefore consider visual summary measures to support
judging the degree of dependence and study their behaviour and
usefulness via a simulation study. Subsequently, the new
multivariate extreme value model is applied to wind data of two
gauging stations where directional aspects are accounted for.
Therefore this model allows for statements about the joint wind
behaviour at the two stations. This knowledge gives insight whether
storm events are likely to be jointly present at larger parts of a
railway track or rather occur localized.
Weitere Episoden

vor 11 Jahren

vor 11 Jahren

vor 11 Jahren
In Podcasts werben
Kommentare (0)