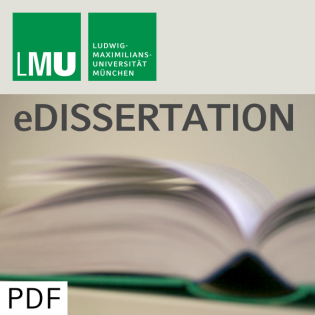
Time-varying coefficient models and measurement error
Beschreibung
vor 17 Jahren
This thesis is concerned with presenting and developing modeling
approaches which allow for a time-varying effect of covariates by
using time-varying coefficients. The different approaches are
compared in simulation studies. Thereby, we investigate how well
different components of the simulated models can be identified. The
models performing best in the simulation study are then applied to
data collected within the study "Improved Air Quality and its
Influences on Short-Term Health Effects in Erfurt, Eastern
Germany". One specific aspect in this analysis is to assess the
necessity of a time-varying estimate compared to a more
parsimonious, time-constant fit. A further topic is the estimation
of time-varying coefficient models in the presence of measurement
errors in the exposure variable. We specify a measurement error
model and present methods to estimate parameters and measurement
error variances of the model in the case of autocorrelated latent
exposure as well as measurement errors. Furthermore, two methods
adjusting for measurement errors in the context of time-varying
coefficients are developed. The first one is based on a
hierarchical Bayesian model and the Bayesian error correction
principle. The second method is an extension of the well-known
regression calibration approach to the case of autocorrelated data.
The obtained estimated true values can then be included into the
main model to assess the effect of the variable of interest.
Finally, the approaches are again applied to the Erfurt data.
approaches which allow for a time-varying effect of covariates by
using time-varying coefficients. The different approaches are
compared in simulation studies. Thereby, we investigate how well
different components of the simulated models can be identified. The
models performing best in the simulation study are then applied to
data collected within the study "Improved Air Quality and its
Influences on Short-Term Health Effects in Erfurt, Eastern
Germany". One specific aspect in this analysis is to assess the
necessity of a time-varying estimate compared to a more
parsimonious, time-constant fit. A further topic is the estimation
of time-varying coefficient models in the presence of measurement
errors in the exposure variable. We specify a measurement error
model and present methods to estimate parameters and measurement
error variances of the model in the case of autocorrelated latent
exposure as well as measurement errors. Furthermore, two methods
adjusting for measurement errors in the context of time-varying
coefficients are developed. The first one is based on a
hierarchical Bayesian model and the Bayesian error correction
principle. The second method is an extension of the well-known
regression calibration approach to the case of autocorrelated data.
The obtained estimated true values can then be included into the
main model to assess the effect of the variable of interest.
Finally, the approaches are again applied to the Erfurt data.
Weitere Episoden

vor 11 Jahren

vor 11 Jahren

vor 11 Jahren
In Podcasts werben
Kommentare (0)