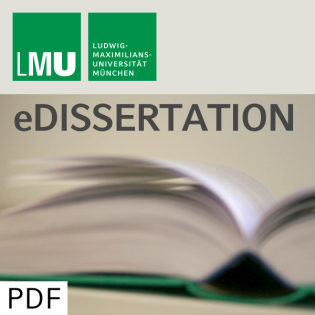
Bioinformatics Methods for NMR Chemical Shift Data
Beschreibung
vor 16 Jahren
Nuclear magnetic resonance spectroscopy (NMR) is one of the most
important methods for measuring the three-dimensional structure of
biomolecules. Despite major progress in the NMR methodology, the
solution of a protein structure is still a tedious and
time-consuming task. The goal of this thesis is to develop
bioinformatics methods which may strongly accelerate the NMR
process. This work concentrates on a special type of measurements,
the so-called chemical shifts. Chemical shifts are routinely
measured at the beginning of a structure resolution process. As all
data from the laboratory, chemical shifts may be error-prone, which
might complicate or even circumvent the use of this data.
Therefore, as the first result of the thesis, we present
CheckShift, a method which automatically corrects a frequent error
in NMR chemical shift data. However, the main goal of this thesis
is the extraction of structural information hidden in chemical
shifts. SimShift was developed as a first step in this direction.
SimShift is the first approach to identify structural similarities
between proteins based on chemical shifts. Compared to methods
based on the amino acid sequence alone, SimShift shows its strength
in detecting distant structural relationships. As a natural further
development of the pairwise comparison of proteins, the SimShift
algorithm is adapted for database searching. Given a protein, the
improved algorithm, named SimShiftDB, searches a database of solved
proteins for structurally homologue entries. The search is based
only on the amino acid sequence and the associated chemical shifts.
The detected similarities are additionally ranked based on
calculations of statistical significance. Finally, the Chemical
Shift Pipeline, the main result of this work, is presented. By
combining automatic chemical shift error correction (CheckShift)
and the database search algorithm (SimShiftDB), it is possible to
achieve very high quality in 70% to 80% of the similarities
identified. Thereby, only about 10% of the predictions are in
error.
important methods for measuring the three-dimensional structure of
biomolecules. Despite major progress in the NMR methodology, the
solution of a protein structure is still a tedious and
time-consuming task. The goal of this thesis is to develop
bioinformatics methods which may strongly accelerate the NMR
process. This work concentrates on a special type of measurements,
the so-called chemical shifts. Chemical shifts are routinely
measured at the beginning of a structure resolution process. As all
data from the laboratory, chemical shifts may be error-prone, which
might complicate or even circumvent the use of this data.
Therefore, as the first result of the thesis, we present
CheckShift, a method which automatically corrects a frequent error
in NMR chemical shift data. However, the main goal of this thesis
is the extraction of structural information hidden in chemical
shifts. SimShift was developed as a first step in this direction.
SimShift is the first approach to identify structural similarities
between proteins based on chemical shifts. Compared to methods
based on the amino acid sequence alone, SimShift shows its strength
in detecting distant structural relationships. As a natural further
development of the pairwise comparison of proteins, the SimShift
algorithm is adapted for database searching. Given a protein, the
improved algorithm, named SimShiftDB, searches a database of solved
proteins for structurally homologue entries. The search is based
only on the amino acid sequence and the associated chemical shifts.
The detected similarities are additionally ranked based on
calculations of statistical significance. Finally, the Chemical
Shift Pipeline, the main result of this work, is presented. By
combining automatic chemical shift error correction (CheckShift)
and the database search algorithm (SimShiftDB), it is possible to
achieve very high quality in 70% to 80% of the similarities
identified. Thereby, only about 10% of the predictions are in
error.
Weitere Episoden

vor 11 Jahren

vor 11 Jahren

vor 11 Jahren
In Podcasts werben
Kommentare (0)