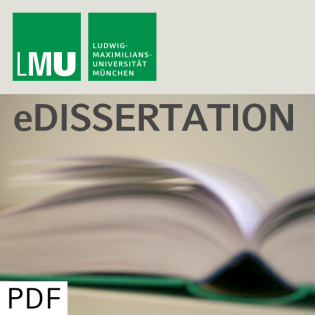
Data-Based Decisions under Complex Uncertainty
Beschreibung
vor 15 Jahren
Decision theory is, in particular in economics, medical expert
systems and statistics, an important tool for determining optimal
decisions under uncertainty. In view of applications in statistics,
the present book is concerned with decision problems which are
explicitly data-based. Since the arising uncertainties are often
too complex to be described by classical precise probability
assessments, concepts of imprecise probabilities (coherent lower
previsions, F-probabilities) are applied. Due to the present state
of research, some basic groundwork has to be done: Firstly,
topological properties of different concepts of imprecise
probabilities are investigated. In particular, the concept of
coherent lower previsions appears to have advantageous properties
for applications in decision theory. Secondly, several decision
theoretic tools are developed for imprecise probabilities. These
tools are mainly based on concepts developed by L. Le Cam and
enable, for example, a definition of sufficiency in case of
imprecise probabilities for the first time. Building on that, the
article [A. Buja, Zeitschrift für Wahrscheinlichkeitstheorie und
Verwandte Gebiete 65 (1984) 367-384] is reinvestigated in the only
recently available framework of imprecise probabilities. This leads
to a generalization of results within the Huber-Strassen theory
concerning least favorable pairs or models. Results obtained by
these investigations can also be applied afterwards in order to
justify the use of the method of natural extension, which is
fundamental within the theory of imprecise probabilities, in
data-based decision problems. It is shown by means of the theory of
vector lattices that applying the method of natural extension in
decision problems does not affect the optimality of decisions.
However, it is also shown that, in general, the method of natural
extension suffers from a severe instability. The book closes with
an application in statistics in which a minimum distance estimator
is developed for imprecise probabilities. After an investigation
concerning its asymptotic properties, an algorithm for calculating
the estimator is given which is based on linear programming. This
algorithm has led to an implementation of the estimator in the
programming language R which is publicly available as R package
"imprProbEst". The applicability of the estimator (even for large
sample sizes) is demonstrated in a simulation study.
systems and statistics, an important tool for determining optimal
decisions under uncertainty. In view of applications in statistics,
the present book is concerned with decision problems which are
explicitly data-based. Since the arising uncertainties are often
too complex to be described by classical precise probability
assessments, concepts of imprecise probabilities (coherent lower
previsions, F-probabilities) are applied. Due to the present state
of research, some basic groundwork has to be done: Firstly,
topological properties of different concepts of imprecise
probabilities are investigated. In particular, the concept of
coherent lower previsions appears to have advantageous properties
for applications in decision theory. Secondly, several decision
theoretic tools are developed for imprecise probabilities. These
tools are mainly based on concepts developed by L. Le Cam and
enable, for example, a definition of sufficiency in case of
imprecise probabilities for the first time. Building on that, the
article [A. Buja, Zeitschrift für Wahrscheinlichkeitstheorie und
Verwandte Gebiete 65 (1984) 367-384] is reinvestigated in the only
recently available framework of imprecise probabilities. This leads
to a generalization of results within the Huber-Strassen theory
concerning least favorable pairs or models. Results obtained by
these investigations can also be applied afterwards in order to
justify the use of the method of natural extension, which is
fundamental within the theory of imprecise probabilities, in
data-based decision problems. It is shown by means of the theory of
vector lattices that applying the method of natural extension in
decision problems does not affect the optimality of decisions.
However, it is also shown that, in general, the method of natural
extension suffers from a severe instability. The book closes with
an application in statistics in which a minimum distance estimator
is developed for imprecise probabilities. After an investigation
concerning its asymptotic properties, an algorithm for calculating
the estimator is given which is based on linear programming. This
algorithm has led to an implementation of the estimator in the
programming language R which is publicly available as R package
"imprProbEst". The applicability of the estimator (even for large
sample sizes) is demonstrated in a simulation study.
Weitere Episoden

vor 11 Jahren

vor 11 Jahren

vor 11 Jahren
In Podcasts werben
Kommentare (0)