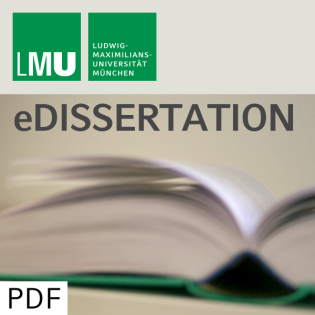
Sequential Dimension Reduction and Prediction Methods with High-dimensional Microarray Data
Beschreibung
vor 15 Jahren
In this thesis, a novel sequential genes selection and
classification (k-SS) method is proposed. The method is analogous
to the classical non-linear stepwise variable selection (SVS)
methods but unlike any of the SVS methods, this new method uses the
misclassification error rates (MERs) as its search criteria for
informative marker genes in any given microarray data. Here, the
importance of any selected gene is determined based on its marginal
contribution at improving the prediction accuracy of the
classification rule. This method ensures continuous selection of
more genes in as much as the improvements brought into the decision
models by the selected genes are considered to be significant
enough by some established test criteria. However, further gene
selection terminates when none of the remaining genes is capable at
improving the prediction accuracy (lowering the MER) of the current
model. Therefore, our approach only seeks to select the best
combination of k marker genes that are most predictive of the
biological samples in any given microarray data sets. An important
feature of our new k-SS method is that the size α used by its test
is not arbitrarily fixed by the user as common to some of the
classical SVS methods. Rather, the value of α at which the best
prediction accuracy is achieved (or the best combination of genes
is selected) is determined by cross-validation. The new k-SS
classifier competes favourably with selected eight existing
classification methods using eleven published microarray data sets.
The k-SS classifier is very simple to apply and does not require
any rigid assumption for its implementation. Another merit of this
method lies in its ability to select only those genes that are of
biological relevance to the existing cancer sub-groups in
microarray data sets. Lastly, we proposed a new preliminary feature
selection procedure that employs the cross-validated area under the
ROC curve (CVAUC) for gene selection. This method is capable at
removing all the irrelevant genes at the preliminary selection
stage before any standard classifier like the k-SS method is
employed on the remaining data set for final optimum gene selection
and classification of mRNA samples. Unlike some other data pruning
methods, the new method employs the sub-sampling technique of the
v-fold cross-validation to ensure consistency and efficiency of
selections made at the preliminary selection stage.
classification (k-SS) method is proposed. The method is analogous
to the classical non-linear stepwise variable selection (SVS)
methods but unlike any of the SVS methods, this new method uses the
misclassification error rates (MERs) as its search criteria for
informative marker genes in any given microarray data. Here, the
importance of any selected gene is determined based on its marginal
contribution at improving the prediction accuracy of the
classification rule. This method ensures continuous selection of
more genes in as much as the improvements brought into the decision
models by the selected genes are considered to be significant
enough by some established test criteria. However, further gene
selection terminates when none of the remaining genes is capable at
improving the prediction accuracy (lowering the MER) of the current
model. Therefore, our approach only seeks to select the best
combination of k marker genes that are most predictive of the
biological samples in any given microarray data sets. An important
feature of our new k-SS method is that the size α used by its test
is not arbitrarily fixed by the user as common to some of the
classical SVS methods. Rather, the value of α at which the best
prediction accuracy is achieved (or the best combination of genes
is selected) is determined by cross-validation. The new k-SS
classifier competes favourably with selected eight existing
classification methods using eleven published microarray data sets.
The k-SS classifier is very simple to apply and does not require
any rigid assumption for its implementation. Another merit of this
method lies in its ability to select only those genes that are of
biological relevance to the existing cancer sub-groups in
microarray data sets. Lastly, we proposed a new preliminary feature
selection procedure that employs the cross-validated area under the
ROC curve (CVAUC) for gene selection. This method is capable at
removing all the irrelevant genes at the preliminary selection
stage before any standard classifier like the k-SS method is
employed on the remaining data set for final optimum gene selection
and classification of mRNA samples. Unlike some other data pruning
methods, the new method employs the sub-sampling technique of the
v-fold cross-validation to ensure consistency and efficiency of
selections made at the preliminary selection stage.
Weitere Episoden

vor 11 Jahren

vor 11 Jahren

vor 11 Jahren
In Podcasts werben
Kommentare (0)