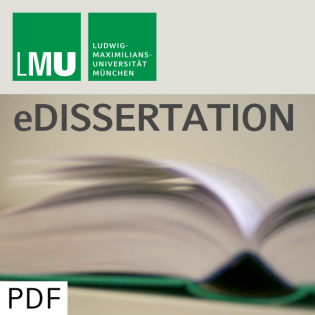
Bayesian Inference for Diffusion Processes with Applications in Life Sciences
Beschreibung
vor 14 Jahren
Diffusion processes are a promising instrument to realistically
model the time-continuous evolution of natural phenomena in life
sciences. However, approximation of a given system is often carried
out heuristically, leading to diffusions that do not correctly
reflect the true dynamics of the original process. Moreover,
statistical inference for diffusions proves to be challenging in
practice as the likelihood function is typically intractable. This
thesis contributes to stochastic modelling and statistical
estimation of real problems in life sciences by means of diffusion
processes. In particular, it creates a framework from existing and
novel techniques for the correct approximation of pure Markov jump
processes by diffusions. Concerning statistical inference, the
thesis reviews existing practices and analyses and further develops
a well-known Bayesian approach which introduces auxiliary
observations by means of Markov chain Monte Carlo (MCMC)
techniques. This procedure originally suffers from convergence
problems which stem from a deterministic link between the model
parameters and the quadratic variation of a continuously observed
diffusion path. This thesis formulates a neat modification of the
above approach for general multi-dimensional diffusions and
provides the mathematical and empirical proof that the
so-constructed MCMC scheme converges. The potential of the newly
developed modelling and estimation methods is demonstrated in two
real-data application studies: the spatial spread of human
influenza in Germany and the in vivo binding behaviour of proteins
in cell nuclei.
model the time-continuous evolution of natural phenomena in life
sciences. However, approximation of a given system is often carried
out heuristically, leading to diffusions that do not correctly
reflect the true dynamics of the original process. Moreover,
statistical inference for diffusions proves to be challenging in
practice as the likelihood function is typically intractable. This
thesis contributes to stochastic modelling and statistical
estimation of real problems in life sciences by means of diffusion
processes. In particular, it creates a framework from existing and
novel techniques for the correct approximation of pure Markov jump
processes by diffusions. Concerning statistical inference, the
thesis reviews existing practices and analyses and further develops
a well-known Bayesian approach which introduces auxiliary
observations by means of Markov chain Monte Carlo (MCMC)
techniques. This procedure originally suffers from convergence
problems which stem from a deterministic link between the model
parameters and the quadratic variation of a continuously observed
diffusion path. This thesis formulates a neat modification of the
above approach for general multi-dimensional diffusions and
provides the mathematical and empirical proof that the
so-constructed MCMC scheme converges. The potential of the newly
developed modelling and estimation methods is demonstrated in two
real-data application studies: the spatial spread of human
influenza in Germany and the in vivo binding behaviour of proteins
in cell nuclei.
Weitere Episoden

vor 11 Jahren

vor 11 Jahren

vor 11 Jahren
In Podcasts werben
Kommentare (0)