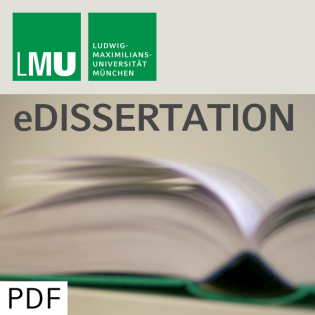
Similarity Search in Medical Data
Beschreibung
vor 13 Jahren
The ongoing automation in our modern information society leads to a
tremendous rise in the amount as well as complexity of collected
data. In medical imaging for example the electronic availability of
extensive data collected as part of clinical trials provides a
remarkable potentiality to detect new relevant features in complex
diseases like brain tumors. Using data mining applications for the
analysis of the data raises several problems. One problem is the
localization of outstanding observations also called outliers in a
data set. In this work a technique for parameter-free outlier
detection, which is based on data compression and a general data
model which combines the Generalized Normal Distribution (GND) with
independent components, to cope with existing problems like
parameter settings or implicit data distribution assumptions, is
proposed. Another problem in many modern applications amongst
others in medical imaging is the efficient similarity search in
uncertain data. At present, an adequate therapy planning of newly
detected brain tumors assumedly of glial origin needs invasive
biopsy due to the fact that prognosis and treatment, both vary
strongly for benign, low-grade, and high-grade tumors. To date
differentiation of tumor grades is mainly based on the expertise of
neuroradiologists examining contrast-enhanced Magnetic Resonance
Images (MRI). To assist neuroradiologist experts during the
differentiation between tumors of different malignancy we proposed
a novel, efficient similarity search technique for uncertain data.
The feature vector of an object is thereby not exactly known but is
rather defined by a Probability Density Function (PDF) like a
Gaussian Mixture Model (GMM). Previous work is limited to
axis-parallel Gaussian distributions, hence, correlations between
different features are not considered in these similarity searches.
In this work a novel, efficient similarity search technique for
general GMMs without independence assumption is presented. The
actual components of a GMM are approximated in a conservative but
tight way. The conservativity of the approach leads to a
filter-refinement architecture, which guarantees no false
dismissals and the tightness of the approximations causes good
filter selectivity. An extensive experimental evaluation of the
approach demonstrates a considerable speed-up of similarity queries
on general GMMs. Additionally, promising results for advancing the
differentiation between brain tumors of different grades could be
obtained by applying the approach to four-dimensional Magnetic
Resonance Images of glioma patients.
tremendous rise in the amount as well as complexity of collected
data. In medical imaging for example the electronic availability of
extensive data collected as part of clinical trials provides a
remarkable potentiality to detect new relevant features in complex
diseases like brain tumors. Using data mining applications for the
analysis of the data raises several problems. One problem is the
localization of outstanding observations also called outliers in a
data set. In this work a technique for parameter-free outlier
detection, which is based on data compression and a general data
model which combines the Generalized Normal Distribution (GND) with
independent components, to cope with existing problems like
parameter settings or implicit data distribution assumptions, is
proposed. Another problem in many modern applications amongst
others in medical imaging is the efficient similarity search in
uncertain data. At present, an adequate therapy planning of newly
detected brain tumors assumedly of glial origin needs invasive
biopsy due to the fact that prognosis and treatment, both vary
strongly for benign, low-grade, and high-grade tumors. To date
differentiation of tumor grades is mainly based on the expertise of
neuroradiologists examining contrast-enhanced Magnetic Resonance
Images (MRI). To assist neuroradiologist experts during the
differentiation between tumors of different malignancy we proposed
a novel, efficient similarity search technique for uncertain data.
The feature vector of an object is thereby not exactly known but is
rather defined by a Probability Density Function (PDF) like a
Gaussian Mixture Model (GMM). Previous work is limited to
axis-parallel Gaussian distributions, hence, correlations between
different features are not considered in these similarity searches.
In this work a novel, efficient similarity search technique for
general GMMs without independence assumption is presented. The
actual components of a GMM are approximated in a conservative but
tight way. The conservativity of the approach leads to a
filter-refinement architecture, which guarantees no false
dismissals and the tightness of the approximations causes good
filter selectivity. An extensive experimental evaluation of the
approach demonstrates a considerable speed-up of similarity queries
on general GMMs. Additionally, promising results for advancing the
differentiation between brain tumors of different grades could be
obtained by applying the approach to four-dimensional Magnetic
Resonance Images of glioma patients.
Weitere Episoden

vor 11 Jahren

vor 11 Jahren

vor 11 Jahren
In Podcasts werben
Kommentare (0)