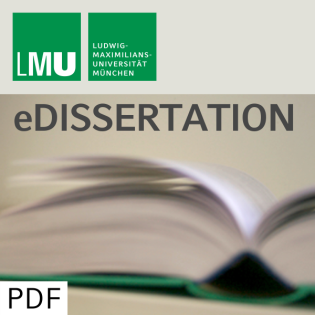
Synchronization Inspired Data Mining
Beschreibung
vor 13 Jahren
Advances of modern technologies produce huge amounts of data in
various fields, increasing the need for efficient and effective
data mining tools to uncover the information contained implicitly
in the data. This thesis mainly aims to propose innovative and
solid algorithms for data mining from a novel perspective:
synchronization. Synchronization is a prevalent phenomenon in
nature that a group of events spontaneously come into co-occurrence
with a common rhythm through mutual interactions. The mechanism of
synchronization allows controlling of complex processes by simple
operations based on interactions between objects. The first main
part of this thesis focuses on developing the innovative algorithms
for data mining. Inspired by the concept of synchronization, this
thesis presents Sync (Clustering by Synchronization), a novel
approach to clustering. In combination with the Minimum Description
Length principle (MDL), it allows discovering the intrinsic
clusters without any data distribution assumptions and parameters
setting. In addition, relying on the dierent dynamic behaviors of
objects during the process towards synchronization,the algorithm
SOD (Synchronization-based Outlier Detection) is further proposed.
The outlier objects can be naturally flagged by the denition of
Local Synchronization Factor (LSF). To cure the curse of
dimensionality in clustering,a subspace clustering algorithm ORSC
is introduced which automatically detects clusters in subspaces of
the original feature space. This approach proposes a weighted local
interaction model to ensure all objects in a common cluster, which
accommodate in arbitrarily oriented subspace, naturally move
together. In order to reveal the underlying patterns in graphs, a
graph partitioning approach RSGC (Robust Synchronization-based
Graph Clustering) is presented. The key philosophy of RSGC is to
consider graph clustering as a dynamic process towards
synchronization. Inherited from the powerful concept of
synchronization, RSGC shows several desirable properties that don't
exist in other competitive methods. For all presented algorithms,
their efficiency and eectiveness are thoroughly analyzed. The
benets over traditional approaches are further demonstrated by
evaluating them on synthetic as well as real-world data sets. Not
only the theory research on novel data mining algorithms, the
second main part of the thesis focuses on brain network analysis
based on Diusion Tensor Images (DTI). A new framework for automated
white matter tracts clustering is rst proposed to identify the
meaningful ber bundles in the Human Brain by combining ideas from
time series mining with density-based clustering. Subsequently, the
enhancement and variation of this approach is discussed allowing
for a more robust, efficient, or eective way to find hierarchies of
ber bundles. Based on the structural connectivity network, an
automated prediction framework is proposed to analyze and
understand the abnormal patterns in patients of Alzheimer's
Disease.
various fields, increasing the need for efficient and effective
data mining tools to uncover the information contained implicitly
in the data. This thesis mainly aims to propose innovative and
solid algorithms for data mining from a novel perspective:
synchronization. Synchronization is a prevalent phenomenon in
nature that a group of events spontaneously come into co-occurrence
with a common rhythm through mutual interactions. The mechanism of
synchronization allows controlling of complex processes by simple
operations based on interactions between objects. The first main
part of this thesis focuses on developing the innovative algorithms
for data mining. Inspired by the concept of synchronization, this
thesis presents Sync (Clustering by Synchronization), a novel
approach to clustering. In combination with the Minimum Description
Length principle (MDL), it allows discovering the intrinsic
clusters without any data distribution assumptions and parameters
setting. In addition, relying on the dierent dynamic behaviors of
objects during the process towards synchronization,the algorithm
SOD (Synchronization-based Outlier Detection) is further proposed.
The outlier objects can be naturally flagged by the denition of
Local Synchronization Factor (LSF). To cure the curse of
dimensionality in clustering,a subspace clustering algorithm ORSC
is introduced which automatically detects clusters in subspaces of
the original feature space. This approach proposes a weighted local
interaction model to ensure all objects in a common cluster, which
accommodate in arbitrarily oriented subspace, naturally move
together. In order to reveal the underlying patterns in graphs, a
graph partitioning approach RSGC (Robust Synchronization-based
Graph Clustering) is presented. The key philosophy of RSGC is to
consider graph clustering as a dynamic process towards
synchronization. Inherited from the powerful concept of
synchronization, RSGC shows several desirable properties that don't
exist in other competitive methods. For all presented algorithms,
their efficiency and eectiveness are thoroughly analyzed. The
benets over traditional approaches are further demonstrated by
evaluating them on synthetic as well as real-world data sets. Not
only the theory research on novel data mining algorithms, the
second main part of the thesis focuses on brain network analysis
based on Diusion Tensor Images (DTI). A new framework for automated
white matter tracts clustering is rst proposed to identify the
meaningful ber bundles in the Human Brain by combining ideas from
time series mining with density-based clustering. Subsequently, the
enhancement and variation of this approach is discussed allowing
for a more robust, efficient, or eective way to find hierarchies of
ber bundles. Based on the structural connectivity network, an
automated prediction framework is proposed to analyze and
understand the abnormal patterns in patients of Alzheimer's
Disease.
Weitere Episoden

vor 11 Jahren

vor 11 Jahren

vor 11 Jahren
In Podcasts werben
Kommentare (0)