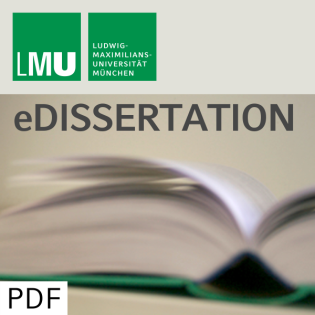
Boosting in structured additive models
Beschreibung
vor 12 Jahren
Variable selection and model choice are of major concern in many
statistical applications, especially in regression models for
high-dimensional data. Boosting is a convenient statistical method
that combines model fitting with intrinsic model selection. We
investigate the impact of base-learner specification on the
performance of boosting as a model selection procedure. We show
that variable selection may be biased if the base-learners have
different degrees of flexibility, both for categorical covariates
and for smooth effects of continuous covariates. We investigate
these problems from a theoretical perspective and suggest a
framework for unbiased model selection based on a general class of
penalized least squares base-learners. Making all base-learners
comparable in terms of their degrees of freedom strongly reduces
the selection bias observed with naive boosting specifications.
Furthermore, the definition of degrees of freedom that is used in
the smoothing literature is questionable in the context of
boosting, and an alternative definition is theoretically derived.
The importance of unbiased model selection is demonstrated in
simulations and in an application to forest health models. A second
aspect of this thesis is the expansion of the boosting algorithm to
new estimation problems: by using constraint base-learners,
monotonicity constrained effect estimates can be seamlessly
incorporated in the existing boosting framework. This holds for
both, smooth effects and ordinal variables. Furthermore, cyclic
restrictions can be integrated in the model for smooth effects of
continuous covariates. In particular in time-series models, cyclic
constraints play an important role. Monotonic and cyclic
constraints of smooth effects can, in addition, be extended to
smooth, bivariate function estimates. If the true effects are
monotonic or cyclic, simulation studies show that constrained
estimates are superior to unconstrained estimates. In three case
studies (the modeling the presence of Red Kite in Bavaria, the
modeling of activity profiles for Roe Deer, and the modeling of
deaths caused by air pollution in Sao Paulo) it is shown that both
constraints can be integrated in the boosting framework and that
they are easy to use. All described results were included in the R
add-on package mboost.
statistical applications, especially in regression models for
high-dimensional data. Boosting is a convenient statistical method
that combines model fitting with intrinsic model selection. We
investigate the impact of base-learner specification on the
performance of boosting as a model selection procedure. We show
that variable selection may be biased if the base-learners have
different degrees of flexibility, both for categorical covariates
and for smooth effects of continuous covariates. We investigate
these problems from a theoretical perspective and suggest a
framework for unbiased model selection based on a general class of
penalized least squares base-learners. Making all base-learners
comparable in terms of their degrees of freedom strongly reduces
the selection bias observed with naive boosting specifications.
Furthermore, the definition of degrees of freedom that is used in
the smoothing literature is questionable in the context of
boosting, and an alternative definition is theoretically derived.
The importance of unbiased model selection is demonstrated in
simulations and in an application to forest health models. A second
aspect of this thesis is the expansion of the boosting algorithm to
new estimation problems: by using constraint base-learners,
monotonicity constrained effect estimates can be seamlessly
incorporated in the existing boosting framework. This holds for
both, smooth effects and ordinal variables. Furthermore, cyclic
restrictions can be integrated in the model for smooth effects of
continuous covariates. In particular in time-series models, cyclic
constraints play an important role. Monotonic and cyclic
constraints of smooth effects can, in addition, be extended to
smooth, bivariate function estimates. If the true effects are
monotonic or cyclic, simulation studies show that constrained
estimates are superior to unconstrained estimates. In three case
studies (the modeling the presence of Red Kite in Bavaria, the
modeling of activity profiles for Roe Deer, and the modeling of
deaths caused by air pollution in Sao Paulo) it is shown that both
constraints can be integrated in the boosting framework and that
they are easy to use. All described results were included in the R
add-on package mboost.
Weitere Episoden

vor 11 Jahren

vor 11 Jahren

vor 11 Jahren
In Podcasts werben
Kommentare (0)