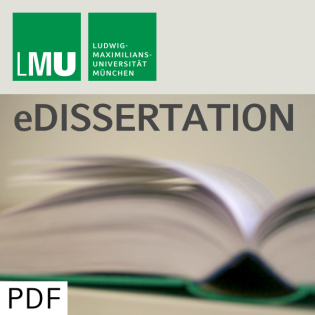
Finding correlations and independences in omics data
Beschreibung
vor 12 Jahren
Biological studies across all omics fields generate vast amounts of
data. To understand these complex data, biologically motivated data
mining techniques are indispensable. Evaluation of the
high-throughput measurements usually relies on the identification
of underlying signals as well as shared or outstanding
characteristics. Therein, methods have been developed to recover
source signals of present datasets, reveal objects which are more
similar to each other than to other objects as well as to detect
observations which are in contrast to the background dataset.
Biological problems got individually addressed by using solutions
from computer science according to their needs. The study of
protein-protein interactions (interactome) focuses on the
identification of clusters, the sub-graphs of graphs: A
parameter-free graph clustering algorithm was developed, which was
based on the concept of graph compression, in order to find sets of
highly interlinked proteins sharing similar characteristics. The
study of lipids (lipidome) calls for co-regulation analyses: To
reveal those lipids similarly responding to biological factors,
partial correlations were generated with differential Gaussian
Graphical Models while accounting for solely disease-specific
correlations. The study on single cell level (cytomics) aims to
understand cellular systems often with the help of microscopy
techniques: A novel noise robust source separation technique
allowed to reliably extract independent components from microscopy
images describing protein behaviors. The study of peptides
(peptidomics) often requires the detection outstanding
observations: By assessing regularities in the data set, an outlier
detection algorithm was implemented based on compression efficacy
of independent components of the dataset. All developed algorithms
had to fulfill most diverse constraints in each omics field, but
were met with methods derived from standard correlation and
dependency analyses.
data. To understand these complex data, biologically motivated data
mining techniques are indispensable. Evaluation of the
high-throughput measurements usually relies on the identification
of underlying signals as well as shared or outstanding
characteristics. Therein, methods have been developed to recover
source signals of present datasets, reveal objects which are more
similar to each other than to other objects as well as to detect
observations which are in contrast to the background dataset.
Biological problems got individually addressed by using solutions
from computer science according to their needs. The study of
protein-protein interactions (interactome) focuses on the
identification of clusters, the sub-graphs of graphs: A
parameter-free graph clustering algorithm was developed, which was
based on the concept of graph compression, in order to find sets of
highly interlinked proteins sharing similar characteristics. The
study of lipids (lipidome) calls for co-regulation analyses: To
reveal those lipids similarly responding to biological factors,
partial correlations were generated with differential Gaussian
Graphical Models while accounting for solely disease-specific
correlations. The study on single cell level (cytomics) aims to
understand cellular systems often with the help of microscopy
techniques: A novel noise robust source separation technique
allowed to reliably extract independent components from microscopy
images describing protein behaviors. The study of peptides
(peptidomics) often requires the detection outstanding
observations: By assessing regularities in the data set, an outlier
detection algorithm was implemented based on compression efficacy
of independent components of the dataset. All developed algorithms
had to fulfill most diverse constraints in each omics field, but
were met with methods derived from standard correlation and
dependency analyses.
Weitere Episoden

vor 11 Jahren

vor 11 Jahren

vor 11 Jahren
In Podcasts werben
Kommentare (0)