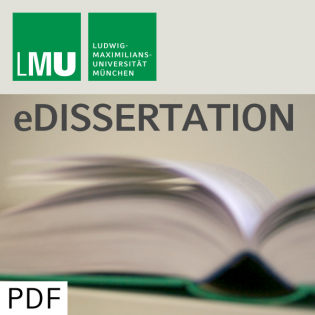
Structured additive quantile regression with applications to modelling undernutrition and obesity of children
Beschreibung
vor 12 Jahren
Quantile regression allows to model the complete conditional
distribution of a response variable - expressed by its quantiles -
depending on covariates, and thereby extends classical regression
models which mainly address the conditional mean of a response
variable. The present thesis introduces the generic model class of
structured additive quantile regression. This model class combines
quantile regression with a structured additive predictor and
thereby enables a variety of covariate effects to be flexibly
modelled. Among other components, the structured additive predictor
comprises smooth non-linear effects of continuous covariates and
individual-specific effects which are particularly important in
longitudinal data settings. Furthermore, this thesis gives an
extensive overview of existing approaches for parameter estimation
in structured additive quantile regression models. These approaches
are structured into distribution-free and distribution-based
approaches as well as related model classes. Each approach is
systematically discussed with regard to the four previously defined
criteria, (i) which different components of the generic predictor
can be estimated, (ii) which properties can be attributed to the
estimators, (iii) if variable selection is possible, and, finally,
(iv) if software is available for practical applications. The main
methodological development of this thesis is a boosting algorithm
which is presented as an alternative estimation approach for
structured additive quantile regression. The discussion of this
innovative approach with respect to the four criteria points out
that quantile boosting involves great advantages regarding almost
all criteria - in particular regarding variable selection. In
addition, the results of several simulation studies provide a
practical comparison of boosting with alternative estimation
approaches. From the beginning of this thesis, the development of
structured additive quantile regression is motivated by two
relevant applications from the field of epidemiology: the
investigation of risk factors for child undernutrition in India (by
a cross-sectional study) and for child overweight and obesity in
Germany (by a birth cohort study). In both applications, extreme
quantiles of the response variables are modelled by structured
additive quantile regression and estimated by quantile boosting.
The results are described and discussed in detail.
distribution of a response variable - expressed by its quantiles -
depending on covariates, and thereby extends classical regression
models which mainly address the conditional mean of a response
variable. The present thesis introduces the generic model class of
structured additive quantile regression. This model class combines
quantile regression with a structured additive predictor and
thereby enables a variety of covariate effects to be flexibly
modelled. Among other components, the structured additive predictor
comprises smooth non-linear effects of continuous covariates and
individual-specific effects which are particularly important in
longitudinal data settings. Furthermore, this thesis gives an
extensive overview of existing approaches for parameter estimation
in structured additive quantile regression models. These approaches
are structured into distribution-free and distribution-based
approaches as well as related model classes. Each approach is
systematically discussed with regard to the four previously defined
criteria, (i) which different components of the generic predictor
can be estimated, (ii) which properties can be attributed to the
estimators, (iii) if variable selection is possible, and, finally,
(iv) if software is available for practical applications. The main
methodological development of this thesis is a boosting algorithm
which is presented as an alternative estimation approach for
structured additive quantile regression. The discussion of this
innovative approach with respect to the four criteria points out
that quantile boosting involves great advantages regarding almost
all criteria - in particular regarding variable selection. In
addition, the results of several simulation studies provide a
practical comparison of boosting with alternative estimation
approaches. From the beginning of this thesis, the development of
structured additive quantile regression is motivated by two
relevant applications from the field of epidemiology: the
investigation of risk factors for child undernutrition in India (by
a cross-sectional study) and for child overweight and obesity in
Germany (by a birth cohort study). In both applications, extreme
quantiles of the response variables are modelled by structured
additive quantile regression and estimated by quantile boosting.
The results are described and discussed in detail.
Weitere Episoden

vor 11 Jahren

vor 11 Jahren

vor 11 Jahren
In Podcasts werben
Kommentare (0)