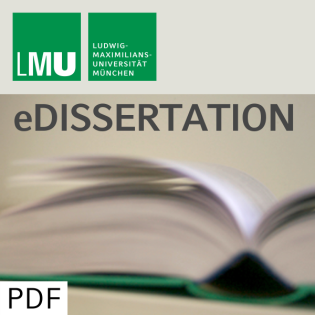
Biostatistical modeling and analysis of combined fMRI and EEG measurements
Beschreibung
vor 12 Jahren
The purpose of brain mapping is to advance the understanding of the
relationship between structure and function in the human brain.
Several techniques---with different advantages and
disadvantages---exist for recording neural activity. Functional
magnetic resonance imaging (fMRI) has a high spatial resolution,
but low temporal resolution. It also suffers from a
low-signal-to-noise ratio in event-related experimental designs,
which are commonly used to investigate neuronal brain activity. On
the other hand, the high temporal resolution of
electroencephalography (EEG) recordings allows to capture provoked
event-related potentials. Though, 3D maps derived by EEG source
reconstruction methods have a low spatial resolution, they provide
complementary information about the location of neuronal activity.
There is a strong interest in combining data from both modalities
to gain a deeper knowledge of brain functioning through advanced
statistical modeling. In this thesis, a new Bayesian method is
proposed for enhancing fMRI activation detection by the use of
EEG-based spatial prior information in stimulus based experimental
paradigms. This method builds upon a newly developed mere fMRI
activation detection method. In general, activation detection
corresponds to stimulus predictor components having an effect on
the fMRI signal trajectory in a voxelwise linear model. We model
and analyze stimulus influence by a spatial Bayesian variable
selection scheme, and extend existing high-dimensional regression
methods by incorporating prior information on binary selection
indicators via a latent probit regression. For mere fMRI activation
detection, the predictor consists of a spatially-varying intercept
only. For EEG-enhanced schemes, an EEG effect is added, which is
either chosen to be spatially-varying or constant.
Spatially-varying effects are regularized by different Markov
random field priors. Statistical inference in resulting
high-dimensional hierarchical models becomes rather challenging
from a modeling perspective as well as with regard to numerical
issues. In this thesis, inference is based on a Markov Chain Monte
Carlo (MCMC) approach relying on global updates of effect maps.
Additionally, a faster algorithm is developed based on single-site
updates to circumvent the computationally intensive,
high-dimensional, sparse Cholesky decompositions. The proposed
algorithms are examined in both simulation studies and real-world
applications. Performance is evaluated in terms of convergency
properties, the ability to produce interpretable results, and the
sensitivity and specificity of corresponding activation
classification rules. The main question is whether the use of EEG
information can increase the power of fMRI models to detect
activated voxels. In summary, the new algorithms show a substantial
increase in sensitivity compared to existing fMRI activation
detection methods like classical SPM. Carefully selected EEG-prior
information additionally increases sensitivity in activation
regions that have been distorted by a low signal-to-noise ratio.
relationship between structure and function in the human brain.
Several techniques---with different advantages and
disadvantages---exist for recording neural activity. Functional
magnetic resonance imaging (fMRI) has a high spatial resolution,
but low temporal resolution. It also suffers from a
low-signal-to-noise ratio in event-related experimental designs,
which are commonly used to investigate neuronal brain activity. On
the other hand, the high temporal resolution of
electroencephalography (EEG) recordings allows to capture provoked
event-related potentials. Though, 3D maps derived by EEG source
reconstruction methods have a low spatial resolution, they provide
complementary information about the location of neuronal activity.
There is a strong interest in combining data from both modalities
to gain a deeper knowledge of brain functioning through advanced
statistical modeling. In this thesis, a new Bayesian method is
proposed for enhancing fMRI activation detection by the use of
EEG-based spatial prior information in stimulus based experimental
paradigms. This method builds upon a newly developed mere fMRI
activation detection method. In general, activation detection
corresponds to stimulus predictor components having an effect on
the fMRI signal trajectory in a voxelwise linear model. We model
and analyze stimulus influence by a spatial Bayesian variable
selection scheme, and extend existing high-dimensional regression
methods by incorporating prior information on binary selection
indicators via a latent probit regression. For mere fMRI activation
detection, the predictor consists of a spatially-varying intercept
only. For EEG-enhanced schemes, an EEG effect is added, which is
either chosen to be spatially-varying or constant.
Spatially-varying effects are regularized by different Markov
random field priors. Statistical inference in resulting
high-dimensional hierarchical models becomes rather challenging
from a modeling perspective as well as with regard to numerical
issues. In this thesis, inference is based on a Markov Chain Monte
Carlo (MCMC) approach relying on global updates of effect maps.
Additionally, a faster algorithm is developed based on single-site
updates to circumvent the computationally intensive,
high-dimensional, sparse Cholesky decompositions. The proposed
algorithms are examined in both simulation studies and real-world
applications. Performance is evaluated in terms of convergency
properties, the ability to produce interpretable results, and the
sensitivity and specificity of corresponding activation
classification rules. The main question is whether the use of EEG
information can increase the power of fMRI models to detect
activated voxels. In summary, the new algorithms show a substantial
increase in sensitivity compared to existing fMRI activation
detection methods like classical SPM. Carefully selected EEG-prior
information additionally increases sensitivity in activation
regions that have been distorted by a low signal-to-noise ratio.
Weitere Episoden

vor 11 Jahren

vor 11 Jahren

vor 11 Jahren
In Podcasts werben
Kommentare (0)