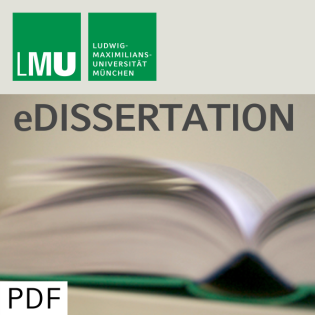
Advances in boosting of temporal and spatial models
Beschreibung
vor 11 Jahren
Boosting is an iterative algorithm for functional approximation and
numerical optimization which can be applied to solve statistical
regression-type problems. By design, boosting can mimic the
solutions of many conventional statistical models, such as the
linear model, the generalized linear model, and the generalized
additive model, but its strength is to enhance these models or even
go beyond. It enjoys increasing attention since a) it is a generic
algorithm, easily extensible to exciting new problems, and b) it
can cope with``difficult'' data where conventional statistical
models fail. In this dissertation, we design autoregressive time
series models based on boosting which capture nonlinearity in the
mean and in the variance, and propose new models for multi-step
forecasting of both. We use a special version of boosting, called
componentwise gradient boosting, which is innovative in the
estimation of the conditional variance of asset returns by sorting
out irrelevant (lagged) predictors. We propose a model which
enables us not only to identify the factors which drive market
volatility, but also to assess the specific nature of their impact.
Therefore, we gain a deeper insight into the nature of the
volatility processes. We analyze four broad asset classes, namely,
stocks, commodities, bonds, and foreign exchange, and use a wide
range of potential macro and financial drivers. The proposed model
for volatility forecasting performs very favorably for stocks and
commodities relative to the common GARCH(1,1) benchmark model. The
advantages are particularly convincing for longer forecasting
horizons. To our knowledge, the application of boosting to
multi-step forecasting of either the mean or the variance has not
been done before. In a separate study, we focus on the conditional
mean of German industrial production. With boosting, we improve the
forecasting accuracy when compared to several competing models
including the benchmark in this field, the linear autoregressive
model. In an exhaustive simulation study we show that boosting of
high-order nonlinear autoregressive time series can be very
competitive in terms of goodness-of-fit when compared to
alternative nonparametric models. Finally, we apply boosting in a
spatio-temporal context to data coming from outside the econometric
field. We estimate the browsing pressure on young beech trees
caused by the game species within the borders of the Bavarian
Forest National Park ``Bayerischer Wald,'' Germany. We found that
using the geographic coordinates of the browsing cases contributes
considerably to the fit. Furthermore, this bivariate geographic
predictor is better suited for prediction if it allows for abrupt
changes in the browsing pressure.
numerical optimization which can be applied to solve statistical
regression-type problems. By design, boosting can mimic the
solutions of many conventional statistical models, such as the
linear model, the generalized linear model, and the generalized
additive model, but its strength is to enhance these models or even
go beyond. It enjoys increasing attention since a) it is a generic
algorithm, easily extensible to exciting new problems, and b) it
can cope with``difficult'' data where conventional statistical
models fail. In this dissertation, we design autoregressive time
series models based on boosting which capture nonlinearity in the
mean and in the variance, and propose new models for multi-step
forecasting of both. We use a special version of boosting, called
componentwise gradient boosting, which is innovative in the
estimation of the conditional variance of asset returns by sorting
out irrelevant (lagged) predictors. We propose a model which
enables us not only to identify the factors which drive market
volatility, but also to assess the specific nature of their impact.
Therefore, we gain a deeper insight into the nature of the
volatility processes. We analyze four broad asset classes, namely,
stocks, commodities, bonds, and foreign exchange, and use a wide
range of potential macro and financial drivers. The proposed model
for volatility forecasting performs very favorably for stocks and
commodities relative to the common GARCH(1,1) benchmark model. The
advantages are particularly convincing for longer forecasting
horizons. To our knowledge, the application of boosting to
multi-step forecasting of either the mean or the variance has not
been done before. In a separate study, we focus on the conditional
mean of German industrial production. With boosting, we improve the
forecasting accuracy when compared to several competing models
including the benchmark in this field, the linear autoregressive
model. In an exhaustive simulation study we show that boosting of
high-order nonlinear autoregressive time series can be very
competitive in terms of goodness-of-fit when compared to
alternative nonparametric models. Finally, we apply boosting in a
spatio-temporal context to data coming from outside the econometric
field. We estimate the browsing pressure on young beech trees
caused by the game species within the borders of the Bavarian
Forest National Park ``Bayerischer Wald,'' Germany. We found that
using the geographic coordinates of the browsing cases contributes
considerably to the fit. Furthermore, this bivariate geographic
predictor is better suited for prediction if it allows for abrupt
changes in the browsing pressure.
Weitere Episoden

vor 11 Jahren

vor 11 Jahren

vor 11 Jahren
In Podcasts werben
Kommentare (0)