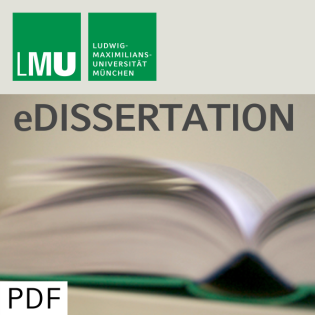
Regularized estimation and model selection in compartment models
Beschreibung
vor 11 Jahren
Dynamic imaging series acquired in medical and biological research
are often analyzed with the help of compartment models. Compartment
models provide a parametric, nonlinear function of interpretable,
kinetic parameters describing how some concentration of interest
evolves over time. Aiming to estimate the kinetic parameters, this
leads to a nonlinear regression problem. In many applications, the
number of compartments needed in the model is not known from
biological considerations but should be inferred from the data
along with the kinetic parameters. As data from medical and
biological experiments are often available in the form of images,
the spatial data structure of the images has to be taken into
account. This thesis addresses the problem of parameter estimation
and model selection in compartment models. Besides a penalized
maximum likelihood based approach, several Bayesian
approaches-including a hierarchical model with Gaussian Markov
random field priors and a model state approach with flexible model
dimension-are proposed and evaluated to accomplish this task.
Existing methods are extended for parameter estimation and model
selection in more complex compartment models. However, in nonlinear
regression and, in particular, for more complex compartment models,
redundancy issues may arise. This thesis analyzes difficulties
arising due to redundancy issues and proposes several approaches to
alleviate those redundancy issues by regularizing the parameter
space. The potential of the proposed estimation and model selection
approaches is evaluated in simulation studies as well as for two in
vivo imaging applications: a dynamic contrast enhanced magnetic
resonance imaging (DCE-MRI) study on breast cancer and a study on
the binding behavior of molecules in living cell nuclei observed in
a fluorescence recovery after photobleaching (FRAP) experiment.
are often analyzed with the help of compartment models. Compartment
models provide a parametric, nonlinear function of interpretable,
kinetic parameters describing how some concentration of interest
evolves over time. Aiming to estimate the kinetic parameters, this
leads to a nonlinear regression problem. In many applications, the
number of compartments needed in the model is not known from
biological considerations but should be inferred from the data
along with the kinetic parameters. As data from medical and
biological experiments are often available in the form of images,
the spatial data structure of the images has to be taken into
account. This thesis addresses the problem of parameter estimation
and model selection in compartment models. Besides a penalized
maximum likelihood based approach, several Bayesian
approaches-including a hierarchical model with Gaussian Markov
random field priors and a model state approach with flexible model
dimension-are proposed and evaluated to accomplish this task.
Existing methods are extended for parameter estimation and model
selection in more complex compartment models. However, in nonlinear
regression and, in particular, for more complex compartment models,
redundancy issues may arise. This thesis analyzes difficulties
arising due to redundancy issues and proposes several approaches to
alleviate those redundancy issues by regularizing the parameter
space. The potential of the proposed estimation and model selection
approaches is evaluated in simulation studies as well as for two in
vivo imaging applications: a dynamic contrast enhanced magnetic
resonance imaging (DCE-MRI) study on breast cancer and a study on
the binding behavior of molecules in living cell nuclei observed in
a fluorescence recovery after photobleaching (FRAP) experiment.
Weitere Episoden

vor 11 Jahren

vor 11 Jahren

vor 11 Jahren
In Podcasts werben
Kommentare (0)