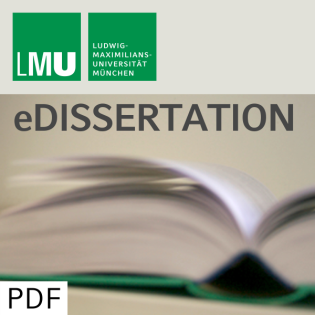
Bayesian regularization in regression models for survival data
Beschreibung
vor 11 Jahren
This thesis is concerned with the development of flexible
continuous-time survival models based on the accelerated failure
time (AFT) model for the survival time and the Cox relative risk
(CRR) model for the hazard rate. The flexibility concerns on the
one hand the extension of the predictor to take into account
simultaneously for a variety of different forms of covariate
effects. On the other hand, the often too restrictive parametric
assumptions about the survival distribution are replaced by
semiparametric approaches that allow very flexible shapes of
survival distribution. We use the Bayesian methodology for
inference. The arising problems, like e. g. the penalization of
high-dimensional linear covariate effects, the smoothing of
nonlinear effects as well as the smoothing of the baseline survival
distribution, are solved with the application of regularization
priors tailored for the respective demand. The considered expansion
of the two survival model classes enables to deal with various
challenges arising in practical analysis of survival data. For
example the models can deal with high-dimensional feature spaces
(e. g. gene expression data), they facilitate feature selection
from the whole set or a subset of the available covariates and
enable the simultaneous modeling of any type of nonlinear covariate
effects for covariates that should always be included in the model.
The option of the nonlinear modeling of covariate effects as well
as the semiparametric modeling of the survival time distribution
enables furthermore also a visual inspection of the linearity
assumptions about the covariate effects or accordingly parametric
assumptions about the survival time distribution. In this thesis it
is shown, how the p>n paradigm, feature relevance,
semiparametric inference for functional effect forms and the
semiparametric inference for the survival distribution can be
treated within a unified Bayesian framework. Due the option to
control the amount of regularization of the considered priors for
the linear regression coefficients, there is no need to distinguish
conceptionally between the cases pn. To accomplish
the desired regularization, the regression coefficients are
associated with shrinkage, selection or smoothing priors. Since the
utilized regularization priors all facilitate a hierarchical
representation, the resulting modular prior structure, in
combination with adequate independence assumptions for the prior
parameters, enables to establish a unified framework and the
possibility to construct efficient MCMC sampling schemes for joint
shrinkage, selection and smoothing in flexible classes of survival
models. The Bayesian formulation enables therefore the simultaneous
estimation of all parameters involved in the models as well as
prediction and uncertainty statements about model specification.
The presented methods are inspired from the flexible and general
approach for structured additive regression (STAR) for responses
from an exponential family and CRR-type survival models. Such
systematic and flexible extensions are in general not available for
AFT models. An aim of this work is to extend the class of AFT
models in order to provide such a rich class of models as resulting
from the STAR approach, where the main focus relies on the
shrinkage of linear effects, the selection of covariates with
linear effects together with the smoothing of nonlinear effects of
continuous covariates as representative of a nonlinear modeling.
Combined are in particular the Bayesian lasso, the Bayesian ridge
and the Bayesian NMIG (a kind of spike-and-slab prior) approach to
regularize the linear effects and the P-spline approach to
regularize the smoothness of the nonlinear effects and the baseline
survival time distribution. To model a flexible error distribution
for the AFT model, the parametric assumption for the baseline error
distribution is replaced by the assumption of a finite Gaussian
mixture distribution. For the special case of specifying one basis
mixture component the estimation problem essentially boils down to
estimation of log-normal AFT model with STAR predictor. In
addition, the existing class of CRR survival models with STAR
predictor, where also baseline hazard rate is approximated by a
P-spline, is expanded to enable the regularization of the linear
effects with the mentioned priors, which broadens further the area
of application of this rich class of CRR models. Finally, the
combined shrinkage, selection and smoothing approach is also
introduced to the semiparametric version of the CRR model, where
the baseline hazard is unspecified and inference is based on the
partial likelihood. Besides the extension of the two survival model
classes the different regularization properties of the considered
shrinkage and selection priors are examined. The developed methods
and algorithms are implemented in the public available software
BayesX and in R-functions and the performance of the methods and
algorithms is extensively tested by simulation studies and
illustrated through three real world data sets.
continuous-time survival models based on the accelerated failure
time (AFT) model for the survival time and the Cox relative risk
(CRR) model for the hazard rate. The flexibility concerns on the
one hand the extension of the predictor to take into account
simultaneously for a variety of different forms of covariate
effects. On the other hand, the often too restrictive parametric
assumptions about the survival distribution are replaced by
semiparametric approaches that allow very flexible shapes of
survival distribution. We use the Bayesian methodology for
inference. The arising problems, like e. g. the penalization of
high-dimensional linear covariate effects, the smoothing of
nonlinear effects as well as the smoothing of the baseline survival
distribution, are solved with the application of regularization
priors tailored for the respective demand. The considered expansion
of the two survival model classes enables to deal with various
challenges arising in practical analysis of survival data. For
example the models can deal with high-dimensional feature spaces
(e. g. gene expression data), they facilitate feature selection
from the whole set or a subset of the available covariates and
enable the simultaneous modeling of any type of nonlinear covariate
effects for covariates that should always be included in the model.
The option of the nonlinear modeling of covariate effects as well
as the semiparametric modeling of the survival time distribution
enables furthermore also a visual inspection of the linearity
assumptions about the covariate effects or accordingly parametric
assumptions about the survival time distribution. In this thesis it
is shown, how the p>n paradigm, feature relevance,
semiparametric inference for functional effect forms and the
semiparametric inference for the survival distribution can be
treated within a unified Bayesian framework. Due the option to
control the amount of regularization of the considered priors for
the linear regression coefficients, there is no need to distinguish
conceptionally between the cases pn. To accomplish
the desired regularization, the regression coefficients are
associated with shrinkage, selection or smoothing priors. Since the
utilized regularization priors all facilitate a hierarchical
representation, the resulting modular prior structure, in
combination with adequate independence assumptions for the prior
parameters, enables to establish a unified framework and the
possibility to construct efficient MCMC sampling schemes for joint
shrinkage, selection and smoothing in flexible classes of survival
models. The Bayesian formulation enables therefore the simultaneous
estimation of all parameters involved in the models as well as
prediction and uncertainty statements about model specification.
The presented methods are inspired from the flexible and general
approach for structured additive regression (STAR) for responses
from an exponential family and CRR-type survival models. Such
systematic and flexible extensions are in general not available for
AFT models. An aim of this work is to extend the class of AFT
models in order to provide such a rich class of models as resulting
from the STAR approach, where the main focus relies on the
shrinkage of linear effects, the selection of covariates with
linear effects together with the smoothing of nonlinear effects of
continuous covariates as representative of a nonlinear modeling.
Combined are in particular the Bayesian lasso, the Bayesian ridge
and the Bayesian NMIG (a kind of spike-and-slab prior) approach to
regularize the linear effects and the P-spline approach to
regularize the smoothness of the nonlinear effects and the baseline
survival time distribution. To model a flexible error distribution
for the AFT model, the parametric assumption for the baseline error
distribution is replaced by the assumption of a finite Gaussian
mixture distribution. For the special case of specifying one basis
mixture component the estimation problem essentially boils down to
estimation of log-normal AFT model with STAR predictor. In
addition, the existing class of CRR survival models with STAR
predictor, where also baseline hazard rate is approximated by a
P-spline, is expanded to enable the regularization of the linear
effects with the mentioned priors, which broadens further the area
of application of this rich class of CRR models. Finally, the
combined shrinkage, selection and smoothing approach is also
introduced to the semiparametric version of the CRR model, where
the baseline hazard is unspecified and inference is based on the
partial likelihood. Besides the extension of the two survival model
classes the different regularization properties of the considered
shrinkage and selection priors are examined. The developed methods
and algorithms are implemented in the public available software
BayesX and in R-functions and the performance of the methods and
algorithms is extensively tested by simulation studies and
illustrated through three real world data sets.
Weitere Episoden

vor 11 Jahren

vor 11 Jahren

vor 11 Jahren
In Podcasts werben
Kommentare (0)