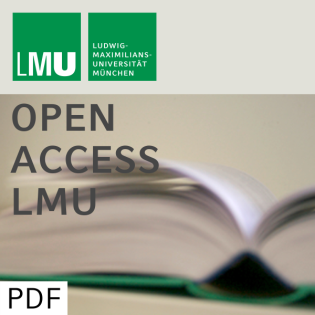
Semiparametric EM-estimation of censored linear regression models for durations
Beschreibung
vor 28 Jahren
This paper investigates the sensitivity of maximum quasi likelihood
estimators of the covariate effects in duration models in the
presence of misspecification due to neglected heterogeneity or
misspecification of the hazard function. We consider linear models
for r(T) where T is duration and r is a known, strictly increasing
function. This class of models is also referred to as
location-scale models. In the absence of censoring, Gould and
Lawless (1988) have shown that maximum likelihood estimators of the
regression parameters are consistent and asymptotically normally
distributed under the assumption that the location-scale structure
of the model is of the correct form. In the presence of censoring,
however, model misspecification leads to inconsistent estimates of
the regression coefficients for most of the censoring mechanisms
that are widely used in practice. We propose a semiparametric
EM-estimator, following ideas of Ritov (1990), and Buckley and
James (1979). This estimator is robust against misspecification and
is highly recommended if there is heavy censoring and if there may
be specification errors. We present the results of simulation
experiments illustrating the performance of the proposed estimator.
estimators of the covariate effects in duration models in the
presence of misspecification due to neglected heterogeneity or
misspecification of the hazard function. We consider linear models
for r(T) where T is duration and r is a known, strictly increasing
function. This class of models is also referred to as
location-scale models. In the absence of censoring, Gould and
Lawless (1988) have shown that maximum likelihood estimators of the
regression parameters are consistent and asymptotically normally
distributed under the assumption that the location-scale structure
of the model is of the correct form. In the presence of censoring,
however, model misspecification leads to inconsistent estimates of
the regression coefficients for most of the censoring mechanisms
that are widely used in practice. We propose a semiparametric
EM-estimator, following ideas of Ritov (1990), and Buckley and
James (1979). This estimator is robust against misspecification and
is highly recommended if there is heavy censoring and if there may
be specification errors. We present the results of simulation
experiments illustrating the performance of the proposed estimator.
Weitere Episoden


In Podcasts werben
Kommentare (0)