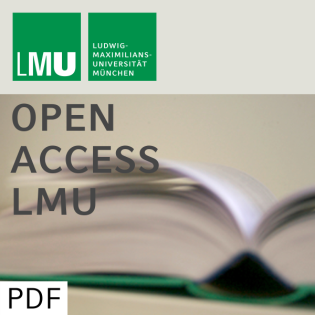
Estimation of Mortalities
Beschreibung
vor 28 Jahren
If a linear regression is fit to log-transformed mortalities and
the estimate is back-transformed according to the formula Ee^Y =
e^{\mu + \sigma^2/2} a systematic bias occurs unless the error
distribution is normal and the scale estimate is gauged to normal
variance. This result is a consequence of the uniqueness theorem
for the Laplace transform. We determine the systematic bias of
minimum-L_2 and minimum-L_1 estimation with sample variance and
interquartile range of the residuals as scale estimates under a
uniform and four contaminated normal error distributions. Already
under innocent looking contaminations the true mortalities may be
underestimated by 50% in the long run. Moreover, the logarithmic
transformation introduces an instability into the model that
results in a large discrepancy between rg_Huber estimates as the
tuning constant regulating the degree of robustness varies.
Contrary to the logarithm the square root stabilizes variance,
diminishes the influence of outliers, automatically copes with
observed zeros, allows the `nonparametric' back-transformation
formula E Y^2 = \mue^2 + \sigma^2, and in the homoskedastic case
avoids a systematic bias of minimum-L_2 estimation with sample
variance. For the company-specific table 3 of [Loeb94], in the age
range of 20-65 years, we fit a parabola to root mortalities by
minimum-L_2 , minimum-L_1, and robust rg_Huber regression
estimates, and a cubic and exponential by least squares. The fits
thus obtained in the original model are excellent and practically
indistinguishable by a \chi^2 goodness-of-fit test. Finally ,
dispensing with the transformation of observations, we employ a
Poisson generalized linear model and fit an exponential and a cubic
by maximum likelihood.
the estimate is back-transformed according to the formula Ee^Y =
e^{\mu + \sigma^2/2} a systematic bias occurs unless the error
distribution is normal and the scale estimate is gauged to normal
variance. This result is a consequence of the uniqueness theorem
for the Laplace transform. We determine the systematic bias of
minimum-L_2 and minimum-L_1 estimation with sample variance and
interquartile range of the residuals as scale estimates under a
uniform and four contaminated normal error distributions. Already
under innocent looking contaminations the true mortalities may be
underestimated by 50% in the long run. Moreover, the logarithmic
transformation introduces an instability into the model that
results in a large discrepancy between rg_Huber estimates as the
tuning constant regulating the degree of robustness varies.
Contrary to the logarithm the square root stabilizes variance,
diminishes the influence of outliers, automatically copes with
observed zeros, allows the `nonparametric' back-transformation
formula E Y^2 = \mue^2 + \sigma^2, and in the homoskedastic case
avoids a systematic bias of minimum-L_2 estimation with sample
variance. For the company-specific table 3 of [Loeb94], in the age
range of 20-65 years, we fit a parabola to root mortalities by
minimum-L_2 , minimum-L_1, and robust rg_Huber regression
estimates, and a cubic and exponential by least squares. The fits
thus obtained in the original model are excellent and practically
indistinguishable by a \chi^2 goodness-of-fit test. Finally ,
dispensing with the transformation of observations, we employ a
Poisson generalized linear model and fit an exponential and a cubic
by maximum likelihood.
Weitere Episoden


In Podcasts werben
Kommentare (0)