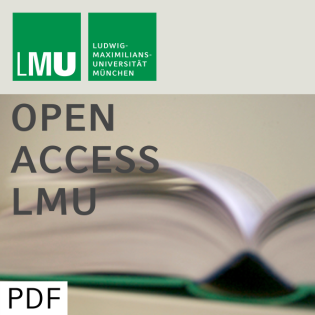
Bayesian spline-type smoothing in generalized regression models
Beschreibung
vor 28 Jahren
Spline smoothing in non- or semiparametric regression models is
usually based on the roughness penalty approach. For regression
with normal errors, the spline smoother also has a Bayesian
justification: Placing a smoothness prior over the regression
function, it is the mean of the posterior given the data. For
non-normal regression this equivalence is lost, but the spline
smoother can still be viewed as the posterior mode. In this paper,
we provide a full Bayesian approach to spline-type smoothing. The
focus is on generalized additive models, however the models can be
extended to other non-normal regression models. Our approach uses
Markov Chain Monte Carlo methods to simulate samples from the
posterior. Thus it is possible to estimate characteristics like the
mean, median, moments, and quantiles of the posterior, or
interesting functionals of the regression function. Also, this
provides an alternative for the choice of smoothing parameters. For
comparison, our approach is applied to real-data examples analyzed
previously by the roughness penalty approach.
usually based on the roughness penalty approach. For regression
with normal errors, the spline smoother also has a Bayesian
justification: Placing a smoothness prior over the regression
function, it is the mean of the posterior given the data. For
non-normal regression this equivalence is lost, but the spline
smoother can still be viewed as the posterior mode. In this paper,
we provide a full Bayesian approach to spline-type smoothing. The
focus is on generalized additive models, however the models can be
extended to other non-normal regression models. Our approach uses
Markov Chain Monte Carlo methods to simulate samples from the
posterior. Thus it is possible to estimate characteristics like the
mean, median, moments, and quantiles of the posterior, or
interesting functionals of the regression function. Also, this
provides an alternative for the choice of smoothing parameters. For
comparison, our approach is applied to real-data examples analyzed
previously by the roughness penalty approach.
Weitere Episoden


In Podcasts werben
Kommentare (0)