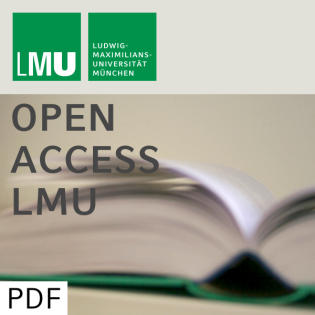
Residual and forecast methods in time series models with covariates
Beschreibung
vor 29 Jahren
We are dealing with time series which are measured on an arbitrary
scale, e.g. on a categorical or ordinal scale, and which are
recorded together with time varying covariates. The conditional
expectations are modelled as a regression model, its parameters are
estimated via likelihood- or quasi-likelihood-approach. Our main
concern are diagnostic methods and forecasting procedures for such
time series models. Diagnostics are based on (partial) residual
measures as well as on (partial) residual variables; l-step
predictors are gained by an approximation formula for conditional
expectations. The various methods proposed are illustrated by two
different data sets.
scale, e.g. on a categorical or ordinal scale, and which are
recorded together with time varying covariates. The conditional
expectations are modelled as a regression model, its parameters are
estimated via likelihood- or quasi-likelihood-approach. Our main
concern are diagnostic methods and forecasting procedures for such
time series models. Diagnostics are based on (partial) residual
measures as well as on (partial) residual variables; l-step
predictors are gained by an approximation formula for conditional
expectations. The various methods proposed are illustrated by two
different data sets.
Weitere Episoden


In Podcasts werben
Abonnenten

"Residual and forecast methods in time series models with covariates"
"Mathematik, Informatik und Statistik - Open Access LMU - Teil 01/03"
15
15
00:00
00:00
"Residual and forecast methods in time series models with covariates"
"Mathematik, Informatik und Statistik - Open Access LMU - Teil 01/03"
Kommentare (0)