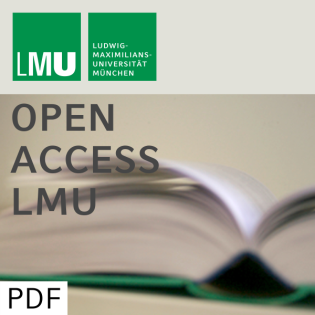
On-line monitoring using Multi-Process Kalman Filtering
Beschreibung
vor 28 Jahren
On-line monitoring of time series becomes more and more important
in different areas of application like medicine, biometry and
finance. In medicine, on-line monitoring of patients after
transplantation of renals (Smith83) is an easy and prominent
example. In finance, fast end reliable recognition of changes in
level and trend of intra-daily stock market prices is of obvious
interest for ordering and purchasing. In this project, we currently
consider monitoring of surgical data like heart-rate, blood
pressure and oxygenation. From a statistical point of view, on-line
monitoring can be considered as on-line detection of changepoints
in time series. That means, changepoints have to be detected in
real time as new observations come in, usually in short time
intervals. Retrospective detection of changepoints, after the whole
batch of observations has been recorded, is nice but useless in
monitoring patients during an operation. There are various
statistical approaches conceivable for on-line detection of
changepoints in time series. Dynamic or state space models seem
particularly well suited because ``filtering'' has historically
been developed exactly for on-line estimation of the ``state'' of
some system. Our approach is based on a recent extension of the
so-called multi-process Kalman filter for changepoint detection
(Schnatter94). It turned out, however, that some important issues
for adequate and reliable application have to be considered, in
particular the (appropriate) handling of outliers and, as a central
point, adaptive on-line estimation of control- or hyper-parameters.
In this paper, we describe a filter model that has this features
and can be implemented in such a way that it is useful for real
time applications with high frequency time series data. Recently,
simulation based methods for estimation of non-Gaussian dynamic
models have been proposed that may also be adapted and generalized
for the purpose of changepoint detection. Most of them solve the
smoothing problem, but very recently some proposals have been made
that could be useful also for filtering and, thus, for on-line
monitoring (Kitagawa96a,Kitagawa96b,Shephard96). If these
approaches are a useful alternative to our development needs a
careful comparison in future and is beyond the scope of this paper.
in different areas of application like medicine, biometry and
finance. In medicine, on-line monitoring of patients after
transplantation of renals (Smith83) is an easy and prominent
example. In finance, fast end reliable recognition of changes in
level and trend of intra-daily stock market prices is of obvious
interest for ordering and purchasing. In this project, we currently
consider monitoring of surgical data like heart-rate, blood
pressure and oxygenation. From a statistical point of view, on-line
monitoring can be considered as on-line detection of changepoints
in time series. That means, changepoints have to be detected in
real time as new observations come in, usually in short time
intervals. Retrospective detection of changepoints, after the whole
batch of observations has been recorded, is nice but useless in
monitoring patients during an operation. There are various
statistical approaches conceivable for on-line detection of
changepoints in time series. Dynamic or state space models seem
particularly well suited because ``filtering'' has historically
been developed exactly for on-line estimation of the ``state'' of
some system. Our approach is based on a recent extension of the
so-called multi-process Kalman filter for changepoint detection
(Schnatter94). It turned out, however, that some important issues
for adequate and reliable application have to be considered, in
particular the (appropriate) handling of outliers and, as a central
point, adaptive on-line estimation of control- or hyper-parameters.
In this paper, we describe a filter model that has this features
and can be implemented in such a way that it is useful for real
time applications with high frequency time series data. Recently,
simulation based methods for estimation of non-Gaussian dynamic
models have been proposed that may also be adapted and generalized
for the purpose of changepoint detection. Most of them solve the
smoothing problem, but very recently some proposals have been made
that could be useful also for filtering and, thus, for on-line
monitoring (Kitagawa96a,Kitagawa96b,Shephard96). If these
approaches are a useful alternative to our development needs a
careful comparison in future and is beyond the scope of this paper.
Weitere Episoden


In Podcasts werben
Kommentare (0)