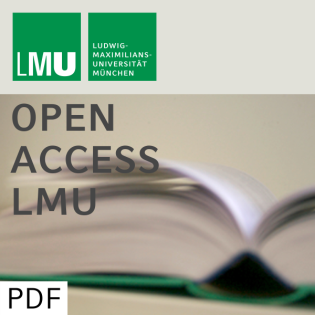
Modelling Count Data with Heteroscedastic Measurement Error in the Covariates
Beschreibung
vor 27 Jahren
This paper is concerned with the estimation of the regression
coefficients for a count data model when one of the explanatory
variables is subject to heteroscedastic measurement error. The
observed values W are related to the true regressor X by the
additive error model W=X+U. The errors U are assumed to be normally
distributed with zero mean but heteroscedastic variances, which are
known or can be estimated from repeated measurements. Inference is
done by using quasi likelihood methods, where a model of the
observed data is specified only through a mean and a variance
function for the response Y given W and other correctly observed
covariates. Although this approach weakens the assumption of a
parametric regression model, there is still the need to determine
the marginal distribution of the unobserved variable X, which is
treated as a random variable. Provided appropriate functions for
the mean and variance are stated, the regression parameters can be
estimated consistently. We illustrate our methods through an
analysis of lung cancer rates in Switzerland. One of the
covariates, the regional radon averages, cannot be measured exactly
due to the strong dependency of radon on geological conditions and
various other environmental sources of influence. The distribution
of the unobserved true radon measure is modelled as a finite
mixture of normals.
coefficients for a count data model when one of the explanatory
variables is subject to heteroscedastic measurement error. The
observed values W are related to the true regressor X by the
additive error model W=X+U. The errors U are assumed to be normally
distributed with zero mean but heteroscedastic variances, which are
known or can be estimated from repeated measurements. Inference is
done by using quasi likelihood methods, where a model of the
observed data is specified only through a mean and a variance
function for the response Y given W and other correctly observed
covariates. Although this approach weakens the assumption of a
parametric regression model, there is still the need to determine
the marginal distribution of the unobserved variable X, which is
treated as a random variable. Provided appropriate functions for
the mean and variance are stated, the regression parameters can be
estimated consistently. We illustrate our methods through an
analysis of lung cancer rates in Switzerland. One of the
covariates, the regional radon averages, cannot be measured exactly
due to the strong dependency of radon on geological conditions and
various other environmental sources of influence. The distribution
of the unobserved true radon measure is modelled as a finite
mixture of normals.
Weitere Episoden


In Podcasts werben
Kommentare (0)